WANG Yan, ZHANG Chenyang, LI Zhaokui. Few-shot hyperspectral image classification based on inter-domain mixup and self-supervised contrastive learning[J]. Laser Journal, 2024, 45(12): 99

Search by keywords or author
- Laser Journal
- Vol. 45, Issue 12, 99 (2024)
Abstract
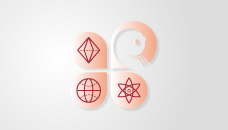
Set citation alerts for the article
Please enter your email address