Hong HUANG, Yichuan YANG, Long WANG, Fujian ZHENG, Jian WU. Boundary Perception Network for Pathological Image Segmentation[J]. Acta Photonica Sinica, 2024, 53(1): 0111003

Search by keywords or author
- Acta Photonica Sinica
- Vol. 53, Issue 1, 0111003 (2024)
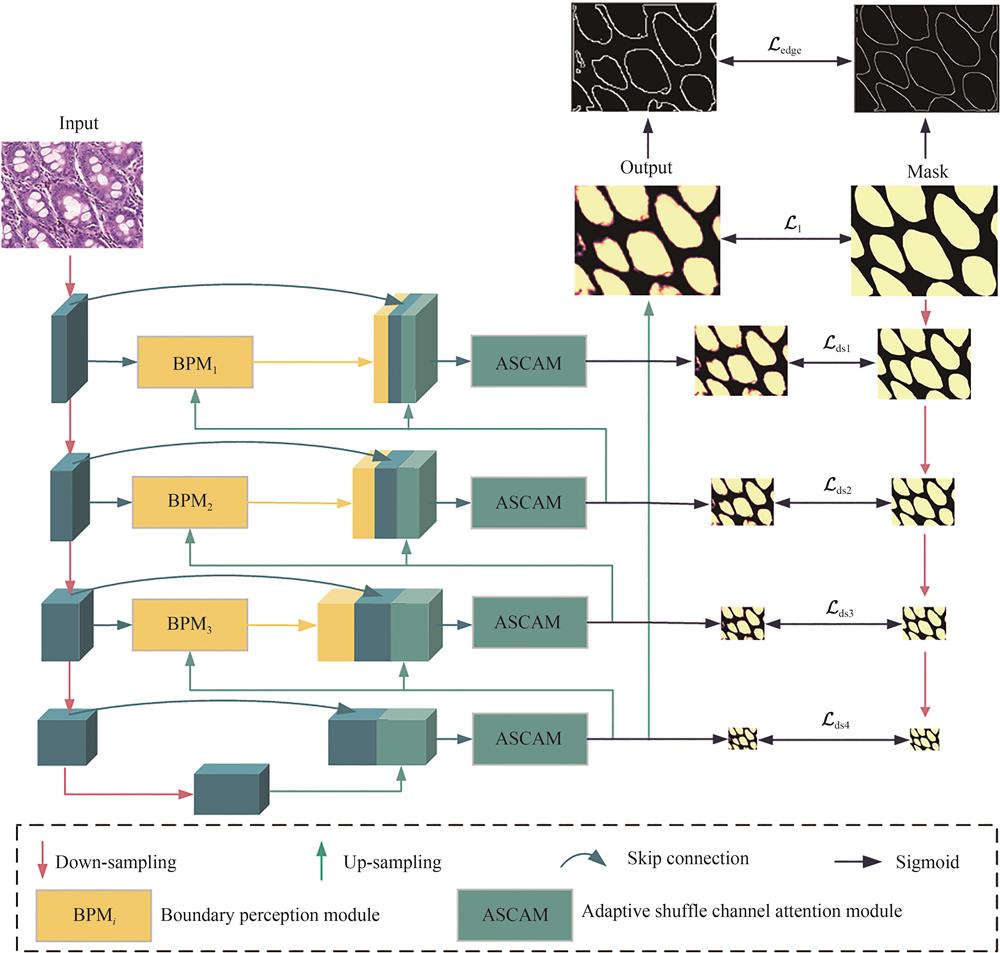
Fig. 1. The overall structure of the BPNet algorithm

Fig. 2. The structure of attention boundary perception module
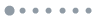
Fig. 3. The structure of adaptive shuffle channel attention module
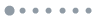
Fig. 4. The GlaS pathological image datasets
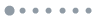
Fig. 5. The MoNuSeg nuclei datasets
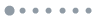
Fig. 6. The segmentation results of different algorithms on GlaS datasets
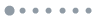
Fig. 7. The segmentation results of ablation experiments on GlaS datasets
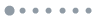
Fig. 8. The segmentation results of different algorithms on MoNuSeg datasets
|
Table 1. The experimental results with different methods on GlaS datasets(Mean ± Std)
|
Table 2. The ablation experimental results on GlaS datasets(Mean ± Std)
|
Table 3. The experimental results with different methods on MoNuSeg datasets(Mean ± Std)
|
Table 4. The ablation experimental results on MoNuSeg datasets(Mean ± Std)
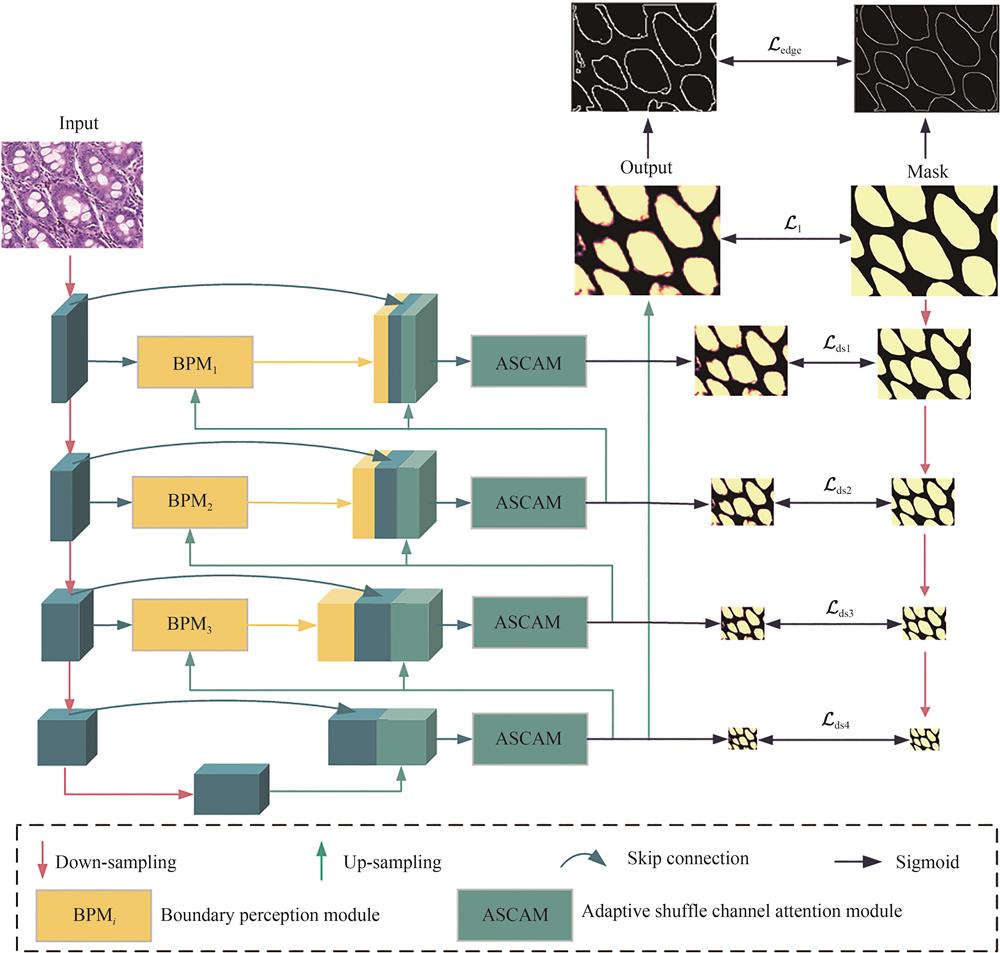
Set citation alerts for the article
Please enter your email address