Lei Xiao, Zongmiao Lan. Identification of Sewage Microorganisms Using Attention Mechanism[J]. Laser & Optoelectronics Progress, 2023, 60(2): 0215002

Search by keywords or author
- Laser & Optoelectronics Progress
- Vol. 60, Issue 2, 0215002 (2023)

Fig. 1. Images of micro-animals. (a) Rotifer; (b) Euplotes; (c) Peranema trichophonrum; (d) Vorticella; (e) Litonotus; (f) Nematode

Fig. 2. Data enhancement effect. (a) Original image; (b) flipping horizontally; (c) rotating 90°; (d) rotating 180°; (e) adding appropriate Gaussian noise
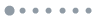
Fig. 3. Squeeze-and-Excitation Network
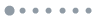
Fig. 4. Comparison images of VGG16 model before and after improvement
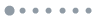
Fig. 5. Training process of improved VGG16 model based on transfer learning
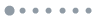
Fig. 6. Accuracy change process on training set
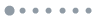
Fig. 7. Accuracy change process on validation set
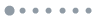
Fig. 8. Loss change process on training set
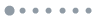
Fig. 9. Loss change process on validation set
|
Table 1. Comparison of number of parameters before and after model improvement
|
Table 2. Comparison of classification performance before and after data augmentation
|
Table 3. Comparison of performance of each model
|
Table 4. Precision of each model
|
Table 5. Recall of each model
|
Table 6. F1-score of each model

Set citation alerts for the article
Please enter your email address