Heng YUAN, Xiaoxue WANG, Tinghao YAN, Shengchong ZHANG. Cross-level feature aggregation image enhancement with dual-path hybrid attention[J]. Optics and Precision Engineering, 2024, 32(10): 1538

Search by keywords or author
- Optics and Precision Engineering
- Vol. 32, Issue 10, 1538 (2024)

Fig. 1. Network structure diagram of this paper

Fig. 2. Structure diagram of MDAR
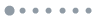
Fig. 3. Structure diagram of DHAB
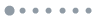
Fig. 4. Structure diagram of CFAM
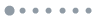
Fig. 5. Visual comparison of different algorithms on the LOL dataset
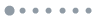
Fig. 6. Visual comparison of different algorithms on the MIT-Adobe 5K dataset
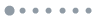
Fig. 7. Ablation experiment visualization of different network modules
|
Table 1. Objective evaluation results of different algorithms on the LOL dataset
|
Table 2. Objective evaluation results of different algorithms on the MIT-Adobe 5K dataset
|
Table 3. Comparison results of evaluation indicators of different network modules
|
Table 4. Average enhancement time of different method

Set citation alerts for the article
Please enter your email address