WANG Teng-jun, ZHAO Ming-hai, YANG Yun, ZHANG Yang, CUI Qin-fang, LI Long-tong. Inversion of Heavy Metals Content in Soil Using Multispectral Remote Sensing Imagery in Daxigou Mining Area of Shaanxi[J]. Spectroscopy and Spectral Analysis, 2019, 39(12): 3880

Search by keywords or author
- Spectroscopy and Spectral Analysis
- Vol. 39, Issue 12, 3880 (2019)
Abstract
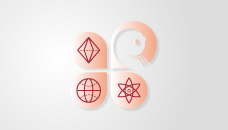
Set citation alerts for the article
Please enter your email address