Hao Wang, Tao Zha, Lingmei Nie, Jun Zhang, Yuxi Tang, Youquan Zhao. Improved Faster R-CNN-Based Contact Lens Surface Defect Detection[J]. Laser & Optoelectronics Progress, 2023, 60(20): 2015004

Search by keywords or author
- Laser & Optoelectronics Progress
- Vol. 60, Issue 20, 2015004 (2023)
Abstract
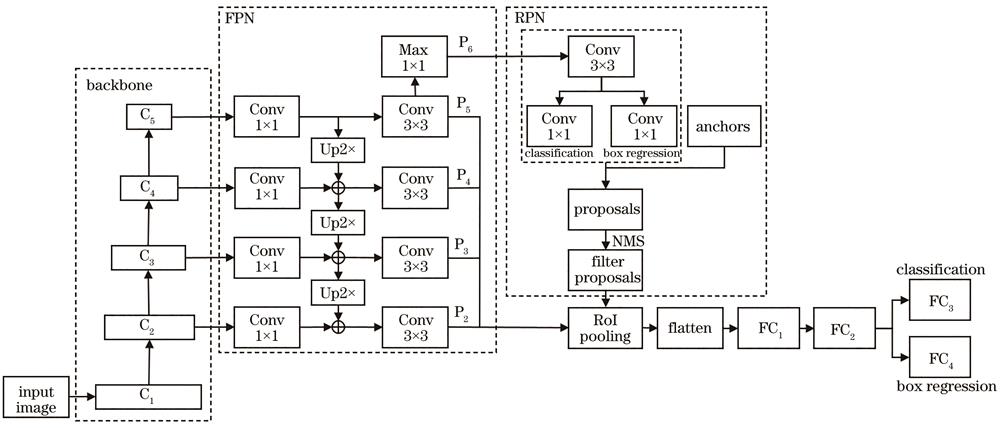
Set citation alerts for the article
Please enter your email address