Ying Li, Yao Dong, Zifen He, Hao Yuan, Fuyang Sun, Lingxi Gong. Defect Detection of Spray Printed Variable Color 2D Code Based on ResNet34-TE[J]. Laser & Optoelectronics Progress, 2024, 61(18): 1812003

Search by keywords or author
- Laser & Optoelectronics Progress
- Vol. 61, Issue 18, 1812003 (2024)
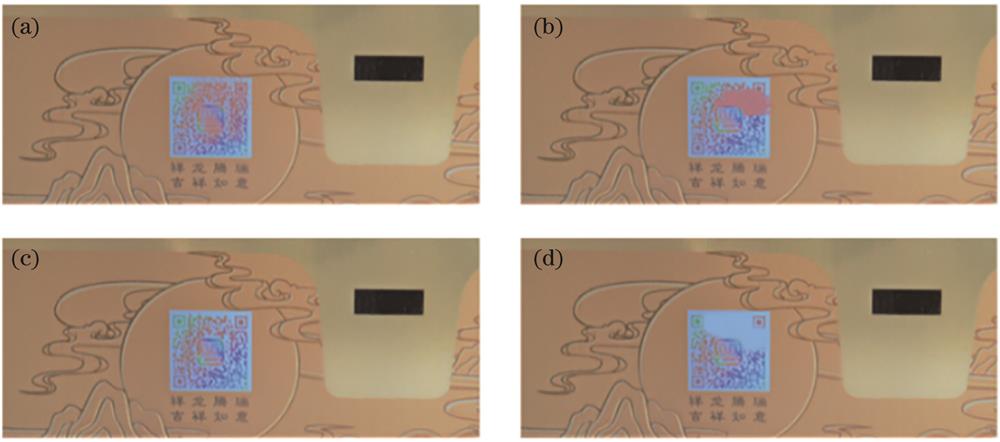
Fig. 1. Defects of color 2D code. (a) Fly-ink defect; (b) paste-ink defect; (c) line defect; (d) omission defect

Fig. 2. Results of different screening conditions
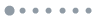
Fig. 3. Extraction process of 2D code area
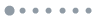
Fig. 4. Structure of residual block
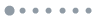
Fig. 5. Framework of the ResNet34-TE model. (a) Overall structure of the model; (b) structure of the residual module of model; (c) structure of Transformer-encoder
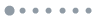
Fig. 6. Process of position embedding
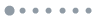
Fig. 7. Structure of encoder. (a) Overall structure of encoder; (b) structure of multi-head attention mechanism; (c) structure of the multilayer perceptron block
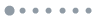
Fig. 8. Flowchart of proposed algorithm
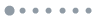
Fig. 9. Comparison of results of different models on validation set. (a) Loss curve; (b) accuracy curve
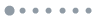
Fig. 10. Confusion matrices of contrasting models. (a) Confusion matrix for ResNet34 model; (b) confusion matrix for ResNet34-TE model
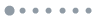
Fig. 11. Prediction results of various defects under different light intensities. (a) Flying ink defect; (b) ink smudging and dirt defect; (c) wire defect; (d) leakage defect
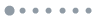
Fig. 12. Visual heat maps of model before and after improvement
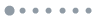
Fig. 13. Sample images of six defects from the NEU-DET dataset
|
Table 1. Dataset distribution
|
Table 2. Comparison of Classification Performance of Classical Models
|
Table 3. Defect classification results of model before and after improvement
|
Table 4. Different light test results
|
Table 5. Results of different encoder block depths
|
Table 6. Results of different models on the NEU-DET dataset
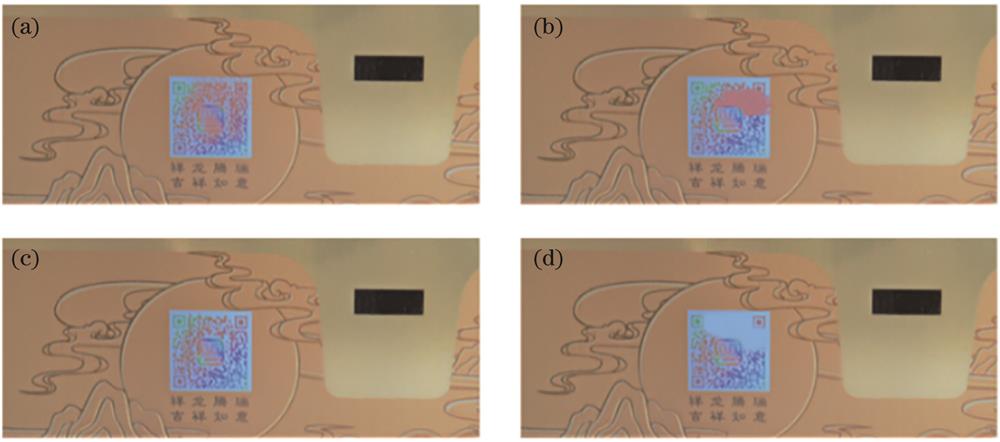
Set citation alerts for the article
Please enter your email address