Yitong Pan, Zhenqi Niu, Songlin Wan, Xiaolin Li, Zhen Cao, Yuying Lu, Jianda Shao, Chaoyang Wei, "Spatial–spectral sparse deep learning combined with a freeform lens enables extreme depth-of-field hyperspectral imaging," Photonics Res. 13, 827 (2025)

Search by keywords or author
- Photonics Research
- Vol. 13, Issue 4, 827 (2025)
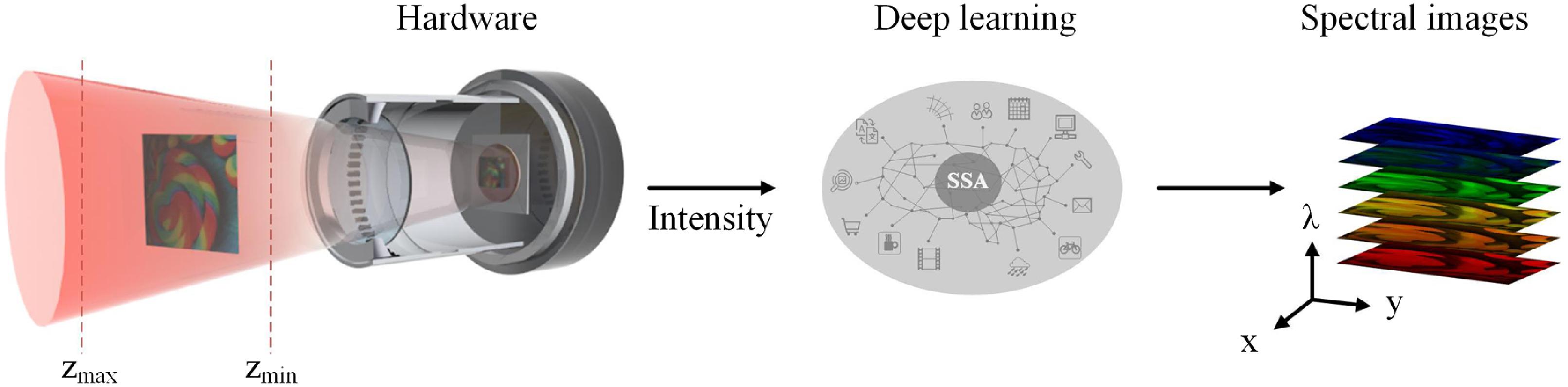
Fig. 1. Proposed E-DoF HI system. The AED hyperspectral images can be regained from the blurred image captured by the camera through subsequent processing by a deep learning neural network with SSA.
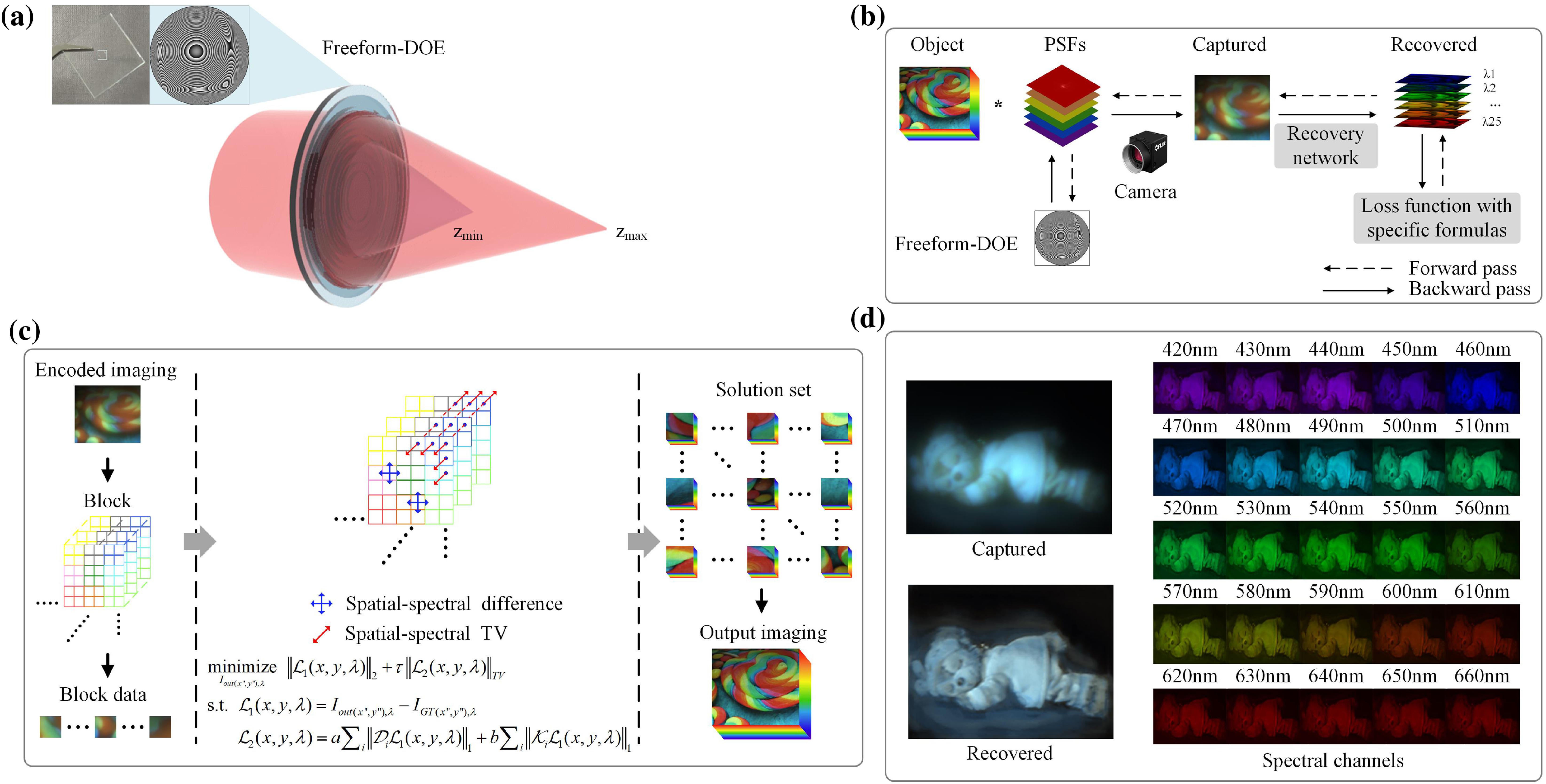
Fig. 2. Overview of AED HI system. (a) Schematic of the proposed system. System allows for high-fidelity imaging across a broad distance from Z min to Z max ; (b) learning pipelines for freeform-DOE. In forward pass, the convolved images of the object and PSFs modulated by the freeform-DOE are captured by a camera and reconstructed by the network. In back pass, the loss function of the recovery network guides the optimization for the freeform-DOE until a satisfactory image can be obtained. (c) Basic framework of SSA. The image is segmented into nine distinct subregions within the 2D plane for processing, each represented by blocks of a unique color. The SSA method is then utilized to limit the difference between neighboring pixels in 2D space and spectral dimension to obtain an optimal output hyperspectral image. (d) Results of hyperspectral reconstruction (illustrated by RGB false color) and its images at different spectral channels.
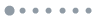
Fig. 3. PSF characteristics of proposed system. (a) PSFs of the system at different object distances. (b) Zoomed-in PSFs at different spectral channels.
![Comparison of simulation results of the three different HI systems considered in the study for the same object at different objective distances d. The images are illustrated by RGB false color (Dataset 1, Ref. [67]).](/Images/icon/loading.gif)
Fig. 4. Comparison of simulation results of the three different HI systems considered in the study for the same object at different objective distances d . The images are illustrated by RGB false color (Dataset 1 , Ref. [67]).
![Experimental results for extended DoF. (a) The experimental scenario diagram. (b) Objects at 1.2–5.2 m from the camera are all in focus; (c) experimental results for extended DoF at a close distance. Objects at 0.5–0.7 m from the camera are also in focus (Dataset 1, Ref. [67]).](/Images/icon/loading.gif)
Fig. 5. Experimental results for extended DoF. (a) The experimental scenario diagram. (b) Objects at 1.2–5.2 m from the camera are all in focus; (c) experimental results for extended DoF at a close distance. Objects at 0.5–0.7 m from the camera are also in focus (Dataset 1 , Ref. [67]).
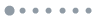
Fig. 6. (a) Visual comparison between proposed system and ground truth (GT). Recovered hyperspectral image and GT are both illustrated by RGB false color. (b) Intensity and accuracy of chosen dots at different wavelengths.
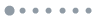
Fig. 7. Experimental results of reconstruction of moving objects. The blocks are pushed down from a height by a pen and their falling process is captured by a camera used in our system. The results of selected moments are shown with the RGB false color and the full results can be found in Visualization 1 .
|
Table 1. Average of SpA of Chosen Dots at Different Wavelengths
|
Table 2. Quantitative Comparison of the State-of-the-Art Methods for E-DoF in Spectral Domain
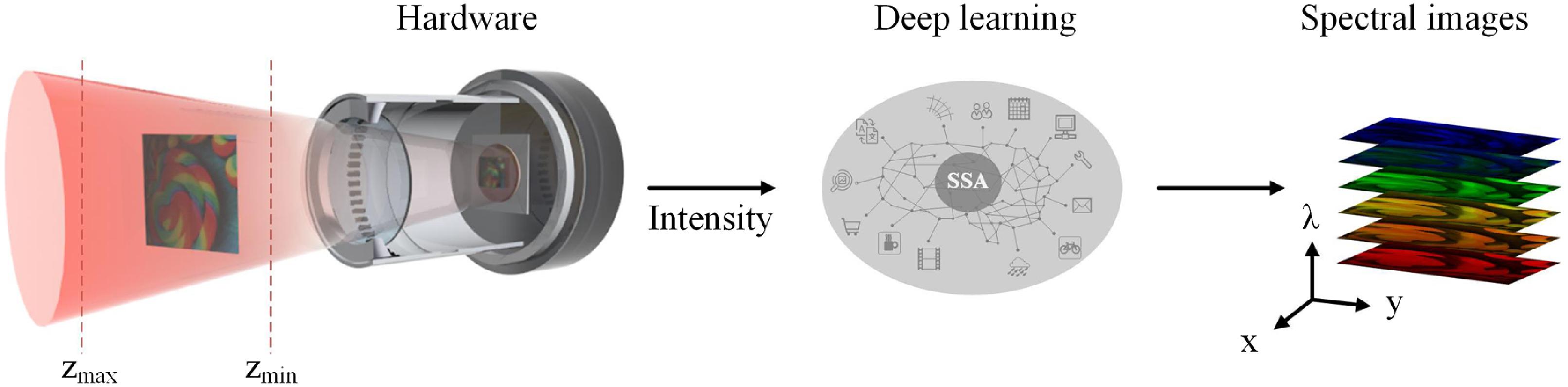
Set citation alerts for the article
Please enter your email address