LIU Shuang, YU Hai-ye, SUI Yuan-yuan, KONG Li-juan, YU Zhan-dong, GUO Jing-jing, QIAO Jian-lei. Hyperspectral Data Analysis for Classification of Soybean Leaf Diseases[J]. Spectroscopy and Spectral Analysis, 2023, 43(5): 1550

Search by keywords or author
- Spectroscopy and Spectral Analysis
- Vol. 43, Issue 5, 1550 (2023)
Abstract
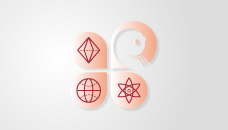
Set citation alerts for the article
Please enter your email address