Jinfu Zhang, Bin Tang, Jianxu Wang, Yanfei Chuan, Zourong Long, Qing Chen, Junfeng Miao, Linfeng Cai, Mingfu Zhao, Mi Zhou. Comparative Analysis of Characteristic Wavelength Screening of Apple Soluble Solids Based on Near-Infrared Spectroscopy[J]. Laser & Optoelectronics Progress, 2023, 60(17): 1730003

Search by keywords or author
- Laser & Optoelectronics Progress
- Vol. 60, Issue 17, 1730003 (2023)

Fig. 1. Schematic diagram of the spectrum acquisition platform
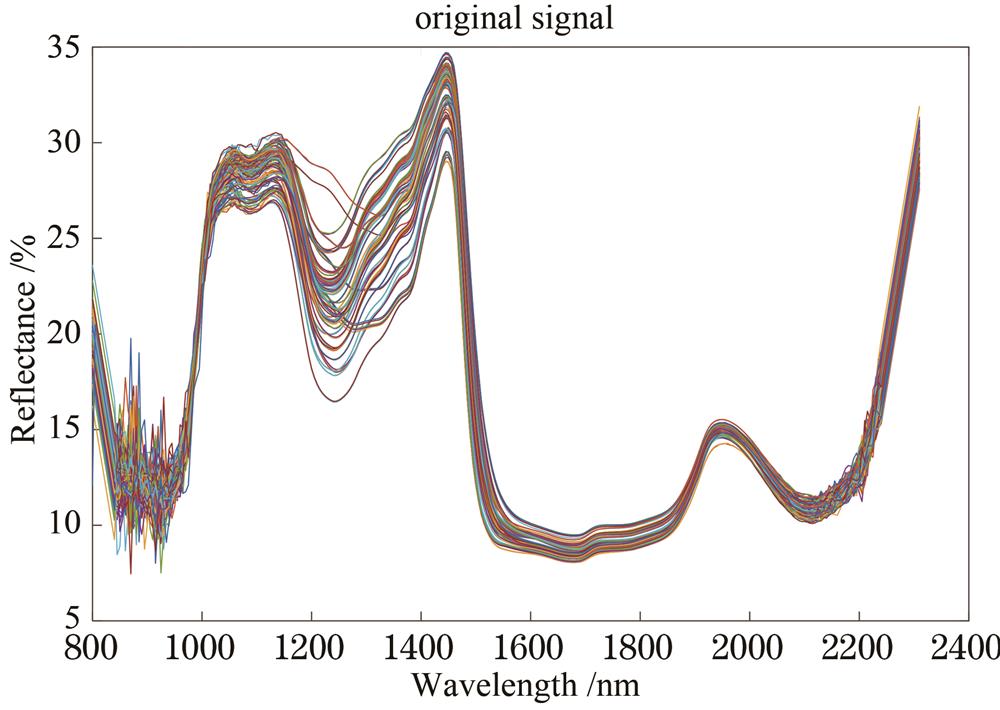
Fig. 2. Original spectra of 120 samples
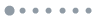
Fig. 3. Comparison of original and preprocessed spectra
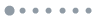
Fig. 4. Flow chart of PLS modeling based on mutual information
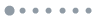
Fig. 5. Relationship between number of PLS principal components and training sets R2 and MSE
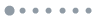
Fig. 6. Full spectrum PLS analysis results
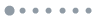
Fig. 7. Screening results of key variables based on CARS. (a) Reserved variables per run; (b) RMSECV of PLS model based on cars; (c) change of variable regression coefficient during each operation, and the asterisk indicates the lowest RMSECV
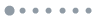
Fig. 8. Analysis of CARS-PLS results
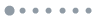
Fig. 9. Analysis of MI-PLS results
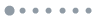
Fig. 10. Selected variables
|
Table 1. Statistics of SSC of 120 samples in the data sets
|
Table 2. SSC prediction results of apple by PLS models using the variables obtained by different variable selection algorithms

Set citation alerts for the article
Please enter your email address