Mengjia Niu, Yongjun Zhang, Zhi Li, Gang Yang, Zhongwei Cui, Junwen Liu. Remote Sensing Image Segmentation Network Based on Adaptive Multiscale and Contour Gradient[J]. Laser & Optoelectronics Progress, 2023, 60(2): 0228009

Search by keywords or author
- Laser & Optoelectronics Progress
- Vol. 60, Issue 2, 0228009 (2023)
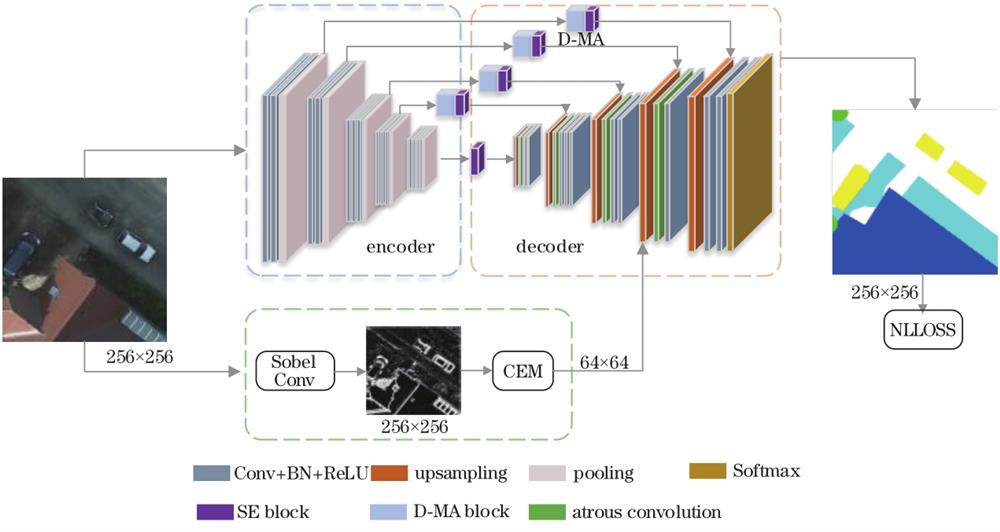
Fig. 1. Framework of remote sensing image semantic segmentation algorithm based on fused contour learning with deep convolutional neural network
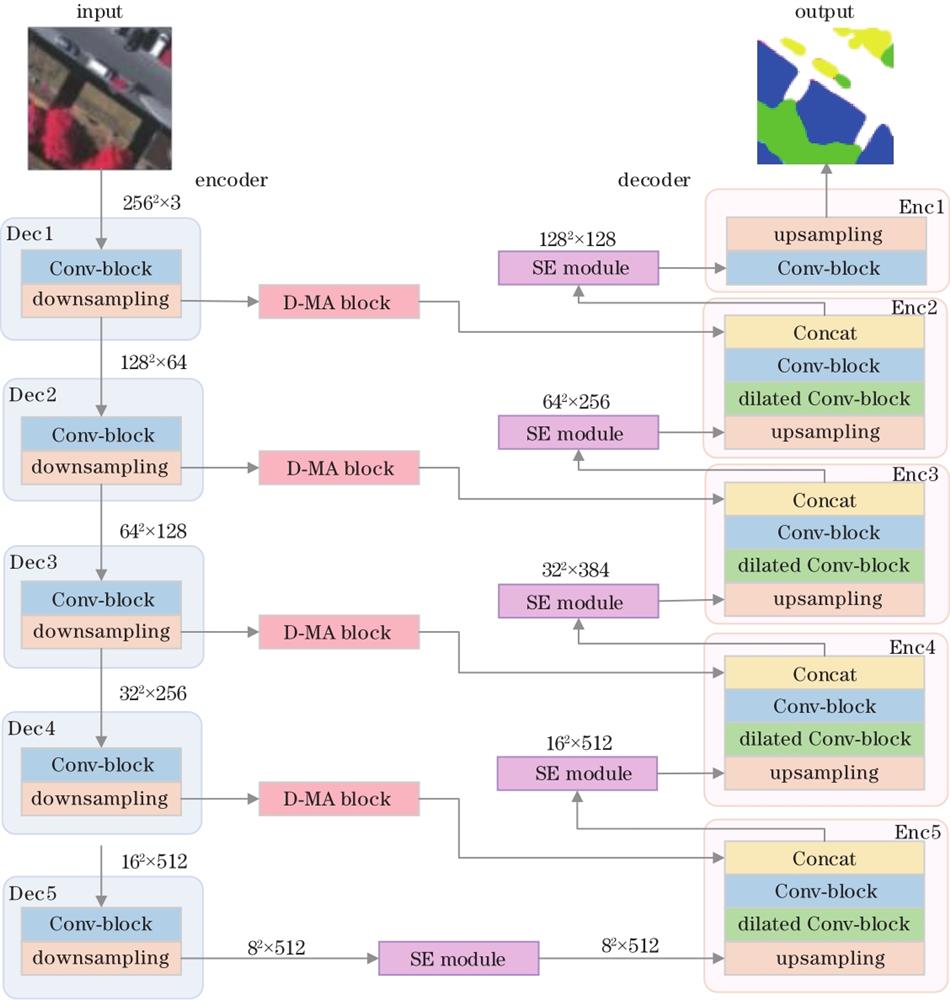
Fig. 2. Multi-channel network framework based on attention mechanism
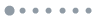
Fig. 3. Diagrams of internal structure of MA block. (a) Internal structure of a single MA block; (b) SK weight module
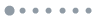
Fig. 4. Diagram of internal structure of D-MA block
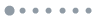
Fig. 5. Visualization of ablation experiments compared in Vaihingen test set
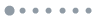
Fig. 6. Comparison of prediction results with mainstream models on Vaihingen test set
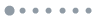
Fig. 7. Comparison of prediction results with mainstream models on Potsdam test set
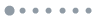
Fig. 8. Comparison of prediction results with mainstream models on WHU building test set
|
Table 1. Configuration parameters for profile extraction module
|
Table 2. Model ablation experiments on Vaihingen dataset
|
Table 3. Comparison with other networks on Vaihingen dataset
|
Table 4. Comparison with other networks on Potsdam dataset
|
Table 5. Comparison with other network indicators on WHU building dataset
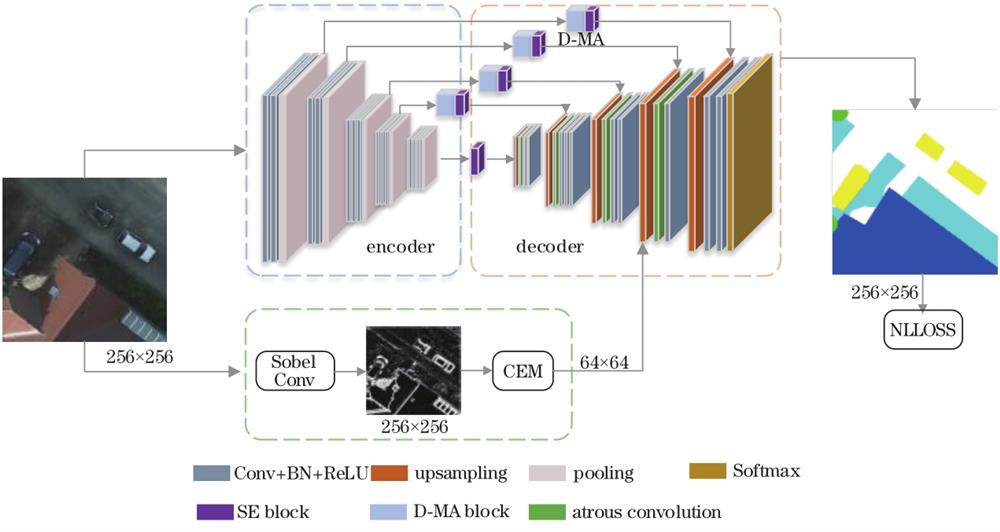
Set citation alerts for the article
Please enter your email address