Qian Wang, Fengdong Chen, Yueyue Han, Fa Zeng, Cheng Lu, Guodong Liu, "Non-blind super-resolution reconstruction for laser-induced damage dark-field imaging of optical elements," Chin. Opt. Lett. 22, 041101 (2024)

Search by keywords or author
- Chinese Optics Letters
- Vol. 22, Issue 4, 041101 (2024)

Fig. 1. Examples of LIDs on an optical element. Images above the dark-field image are the corresponding LIDs captured by a microscope.

Fig. 2. Principle of the multichannel and multifrequency mixing deconvolution method.
![Workflow of the PSF measurement algorithm[12].](/Images/icon/loading.gif)
Fig. 3. Workflow of the PSF measurement algorithm[12].
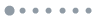
Fig. 4. Principle of the PSF measurement considering the inconsistency at different positions and slight defocus.
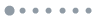
Fig. 5. Sketch of the MMFDNet network structure.
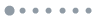
Fig. 6. Measured PSFs in typical position (d = 255 mm).
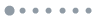
Fig. 7. SR reconstruction results. The first column shows the low-resolution sample input images; the second column shows the high-resolution GT images; the third column shows the SR results of NAFNet; the fourth column shows the SR results of MMFDNet (ours). The data on the image are the PSNR (in dB)/SSIM values.
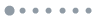
Fig. 8. Result of the adaptive mean threshold segmentation that is used as the damage attention region.
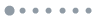
Fig. 9. Search results for the ω. The black dashed line indicates the results of training using the Loss1 function on both PSNR and SSIM values. Other lines represent PSNR and SSIM values when ω takes different values.
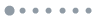
Fig. 10. Comparison of the SR results on low-resolution images acquired by actual cameras.
|
Table 1. Results of NAFNet and MMFDNet (ours) of Partial Samples
|
Table 2. Performance of the Networks and Loss Functions on the Data Set

Set citation alerts for the article
Please enter your email address