Jinming Wang, Peng Li, Yan Liang, Wei Sun, Jie Song, Yadong Feng, Lingxiao Zhao. Esophageal Squamous Cell Carcinoma Recognition Based on Lightweight Residual Networks with an Attention Mechanism[J]. Laser & Optoelectronics Progress, 2023, 60(10): 1010023

Search by keywords or author
- Laser & Optoelectronics Progress
- Vol. 60, Issue 10, 1010023 (2023)

Fig. 1. Architecture of CALite-ResNet
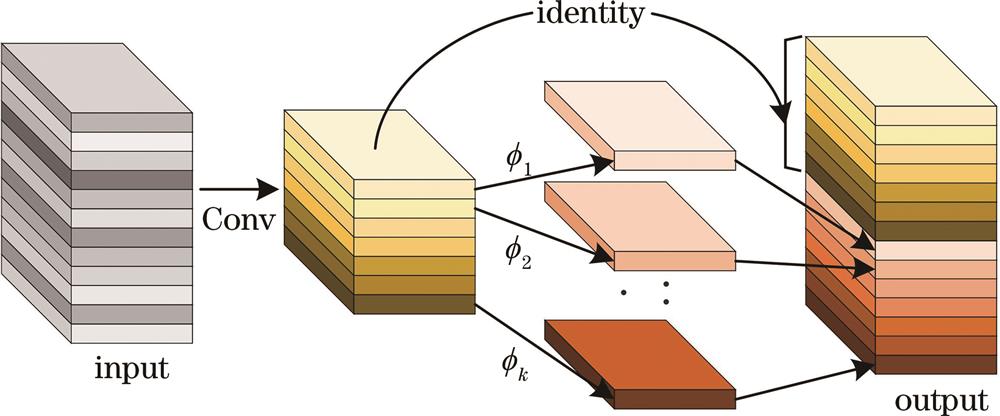
Fig. 2. Schematic of GhostModule
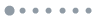
Fig. 3. Schematic illustration of improved SCConv
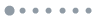
Fig. 4. Diagrams of residual block structures. (a) Bottleneck structure of ResNet50; (b) GSCBottleneck structure
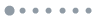
Fig. 5. Structure of CA attention mechanism
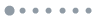
Fig. 6. Structure of CA-GSCBottleneck module
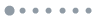
Fig. 7. Schematic of majority voting method
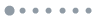
Fig. 8. Example diagrams of effects obtained using different data enhancement methods. (a) Origin image; (b) random rotation; (c) horizontal flip; (d) random scaling
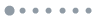
Fig. 9. ROC curves of different network models
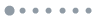
Fig. 10. Grad-CAM visualizations
|
Table 1. Classification results of 5-fold cross-validation at image level
|
Table 2. Classification results of 5-fold cross-validation at patient level
|
Table 3. Comprehensive performance comparison of different network models
|
Table 4. Comparison with related research methods
|
Table 5. Comparison of the results of ablation experiments
|
Table 6. Comparison of experimental results in different attention modules

Set citation alerts for the article
Please enter your email address