XIE Guobo, HE Lin, LIN Zhiyi, ZHANG Wenliang, CHEN Yi. Lightweight optical remote sensing image road extraction based on L-DeepLabv3+[J]. Laser Journal, 2024, 45(3): 111

Search by keywords or author
- Laser Journal
- Vol. 45, Issue 3, 111 (2024)
Abstract
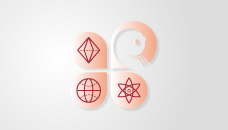
Set citation alerts for the article
Please enter your email address