Zixiang ZHOU, Dandan HUANG, Zhi LIU. Point cloud registration algorithm based on 3D shape context features[J]. Journal of Applied Optics, 2023, 44(2): 330

Search by keywords or author
- Journal of Applied Optics
- Vol. 44, Issue 2, 330 (2023)

Fig. 1. Flow chart of proposed algorithm

Fig. 2. Comparison of point cloud before and after voxel filtering
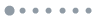
Fig. 3. Comparison results of three commonly-used point cloud feature extraction methods
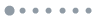
Fig. 4. Diagram of feature space division of 3DSC
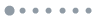
Fig. 5. Matching results of bunny
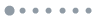
Fig. 6. Matching results of fr1_desk
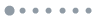
Fig. 7. Matching results of fr2_desk
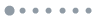
Fig. 8. Matching results of fr2_xyz
|
Table 1. The number of feature points extracted by different methods
|
Table 2. The number of feature points extracted by ISS algorithm under different search radii
|
Table 3. Other main parameters of proposed algorithm
|
Table 4. Number of point clouds and matching of feature points
|
Table 5. Evaluation of registration effect of bunny
|
Table 6. Evaluation of registration effect of fr1_desk
|
Table 7. Evaluation of registration effect of fr2_desk
|
Table 8. Evaluation of registration effect of fr2_xyz

Set citation alerts for the article
Please enter your email address