Sijun DONG, Xiaoliang MENG. Text-Semantics-Driven Feature Extraction from Remote Sensing Imagery[J]. Spacecraft Recovery & Remote Sensing, 2024, 45(3): 82

Search by keywords or author
- Spacecraft Recovery & Remote Sensing
- Vol. 45, Issue 3, 82 (2024)
Note: This section is automatically generated by AI . The website and platform operators shall not be liable for any commercial or legal consequences arising from your use of AI generated content on this website. Please be aware of this.
Abstract
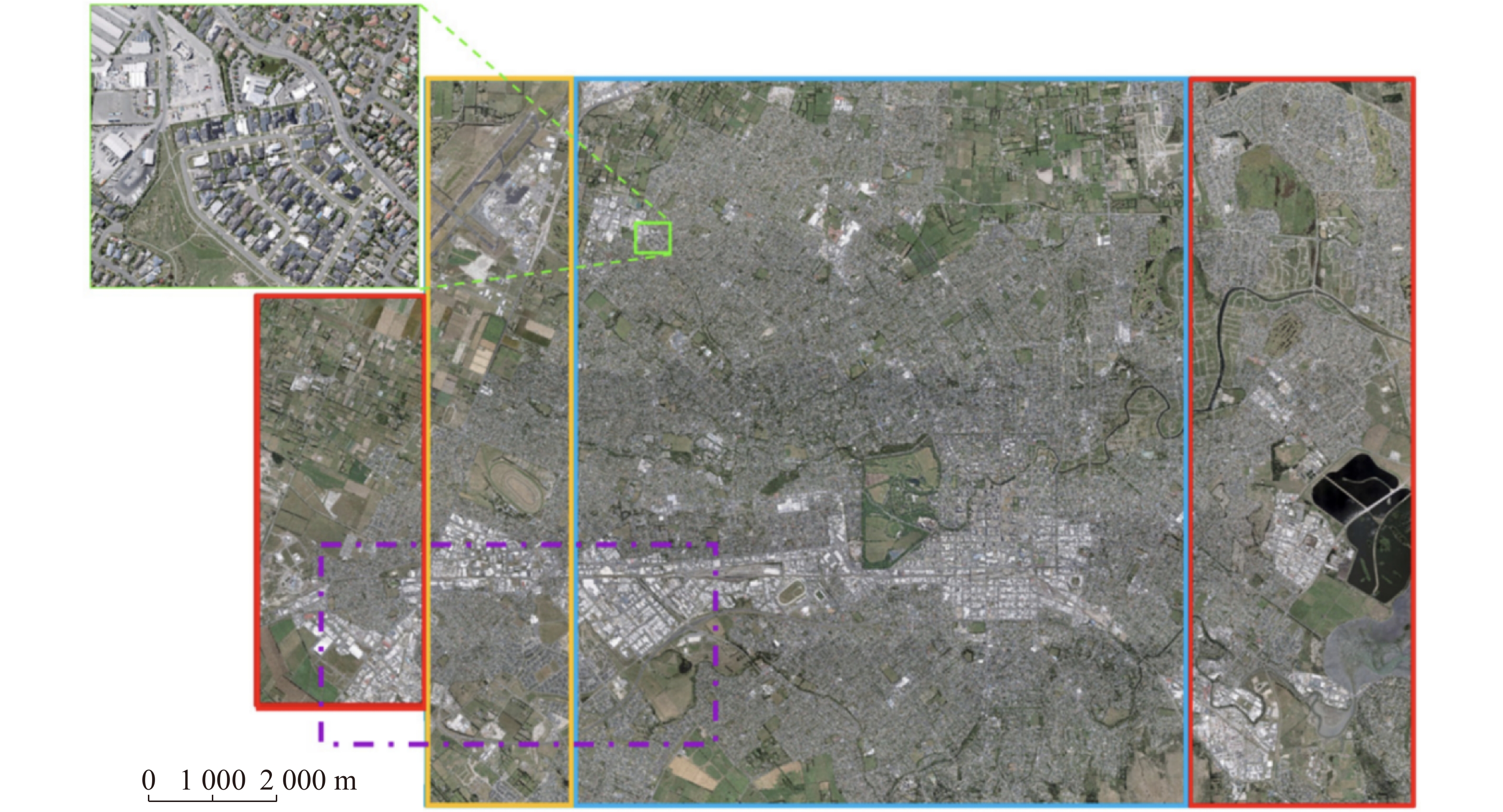
Set citation alerts for the article
Please enter your email address