Fusheng Li, Xiaolong Zeng. Quantitative Analysis Method of Soil Elements Combining Sensitivity Dimensionality Reduction and Support Vector Regression[J]. Laser & Optoelectronics Progress, 2023, 60(5): 0530002

Search by keywords or author
- Laser & Optoelectronics Progress
- Vol. 60, Issue 5, 0530002 (2023)

Fig. 1. Spectra before and after background subtraction. (a) Original spectrum and estimated background; (b) original spectrum and corrected spectrum

Fig. 2. Preprocessing effect after background subtraction
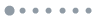
Fig. 3. Flow chart of quantitative analysis method based on BOA-SVR
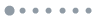
Fig. 4. Physical image of the sample and XRF spectrometer. (a) Sample; (b) XRF spectrometer
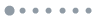
Fig. 5. Sensitivity analysis result of the As element
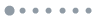
Fig. 6. Prediction results of the model under different feature dimensions
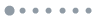
Fig. 7. Prediction results of Cu element. (a) SVR model with feature dimension reduction; (b) SVR model with all features as inputs; (c) PLS model
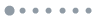
Fig. 8. Prediction results of As element. (a) SVR model with feature dimension reduction; (b) SVR model with all features as inputs; (c) PLS model
|
Table 1. Prediction results of three models on verification set in Cu element verification
|
Table 2. Cu element prediction results obtained by three models
|
Table 3. As element prediction results obtained by three models

Set citation alerts for the article
Please enter your email address