ZHAO Chun-Yang, ZHAO Huai-Ci. Multimodality robust local feature descriptors[J]. Optics and Precision Engineering, 2015, 23(5): 1474

Search by keywords or author
- Optics and Precision Engineering
- Vol. 23, Issue 5, 1474 (2015)
Abstract
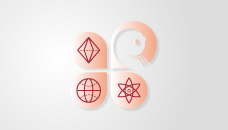
Set citation alerts for the article
Please enter your email address