Wenyue Hao, Huaiyu Cai, Tingtao Zuo, Zhongwei Jia, Yi Wang, Xiaodong Chen. Self-Supervised Pre-Training for Intravascular Ultrasound Image Segmentation Method Based on Diffusion Model[J]. Laser & Optoelectronics Progress, 2024, 61(18): 1837005

Search by keywords or author
- Laser & Optoelectronics Progress
- Vol. 61, Issue 18, 1837005 (2024)
Abstract
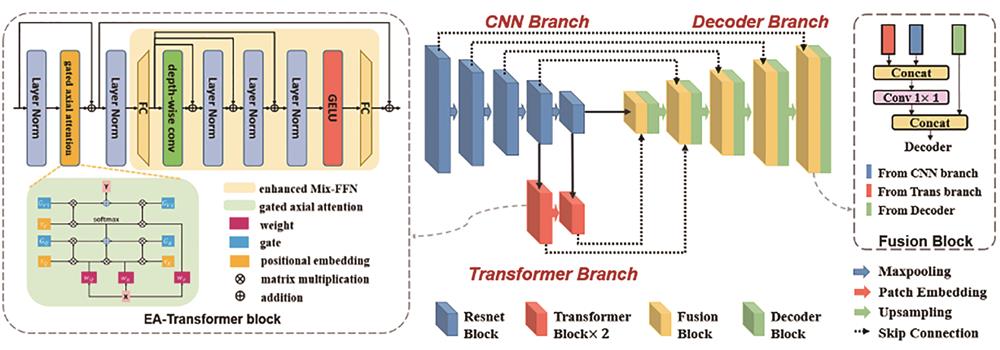
Set citation alerts for the article
Please enter your email address