Guoming YUAN, Guang YANG, Jinfeng WANG, Haijun LIU, Wei WANG. Coarse-to-fine underwater image enhancement based on multi-level wavelet transform[J]. Optics and Precision Engineering, 2022, 30(22): 2939

Search by keywords or author
- Optics and Precision Engineering
- Vol. 30, Issue 22, 2939 (2022)
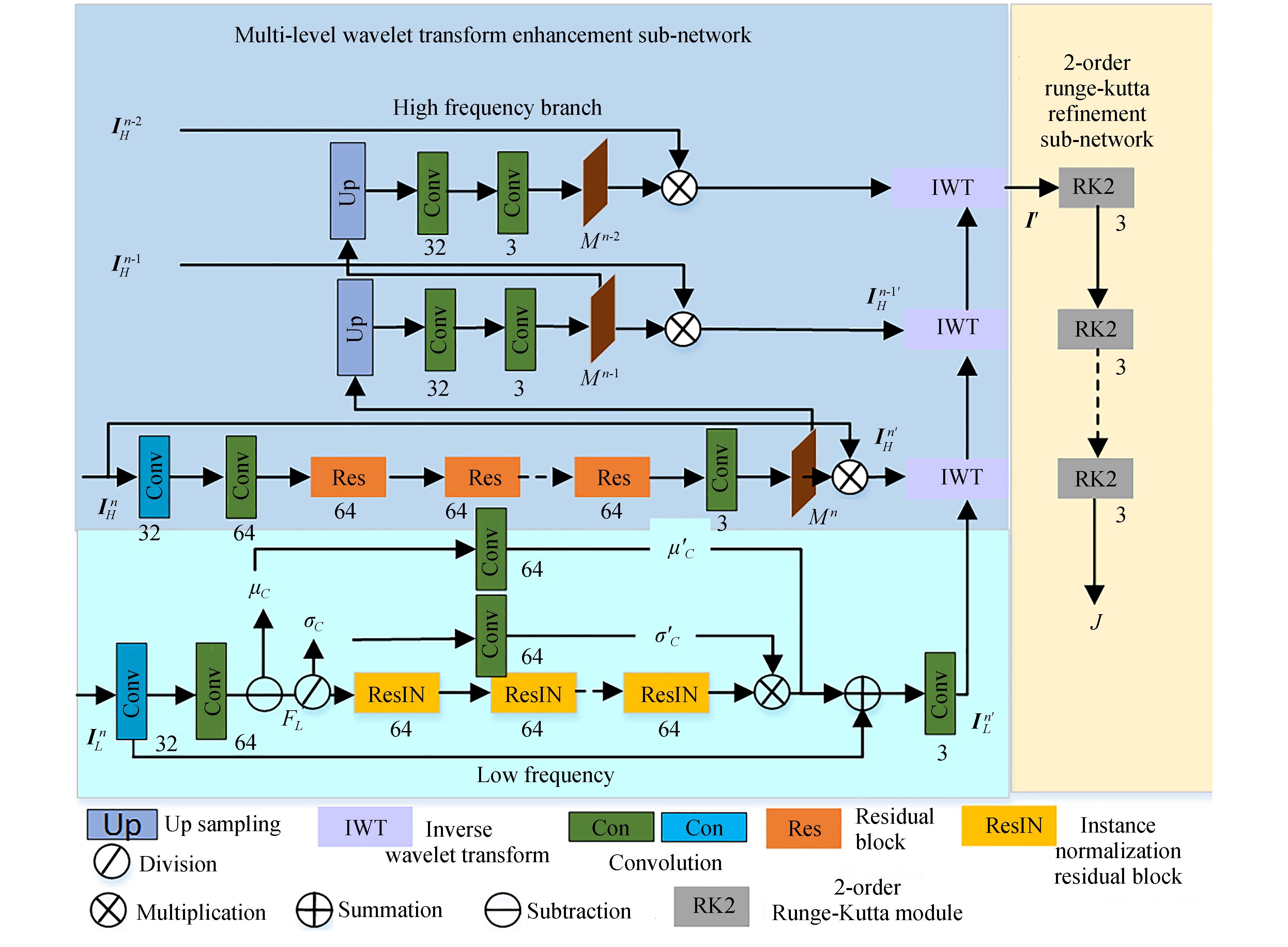
Fig. 1. Architecture of coarse-to-fine network for underwater image enhancement based on multi-level wavelet transform

Fig. 2. Comparison between initial images and enhanced images
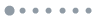
Fig. 3. High frequency image obtained by wavelet transform at different levels
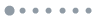
Fig. 4. Architecture of RK2 block
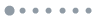
Fig. 5. Loss curves during training.
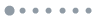
Fig. 6. Average PSNR of testing data during training.
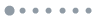
Fig. 7. Testing samples
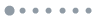
Fig. 8. Comparison of enhanced results on synthetic underwater images
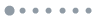
Fig. 9. Comparison of enhanced results on real underwater images
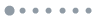
Fig. 10. PSNR of different algorithms on Test1 and Test2.
|
Table 1. Comparison of quantitative results by variant models with different number of building modules on Test 1.
|
Table 2. Comparison of quantitative results by different variant models on Test1.
|
Table 3. Quantitative comparison of enhanced results by different algorithms on Test1.
|
Table 4. Quantitative comparison of enhanced results by different algorithms on Test2.
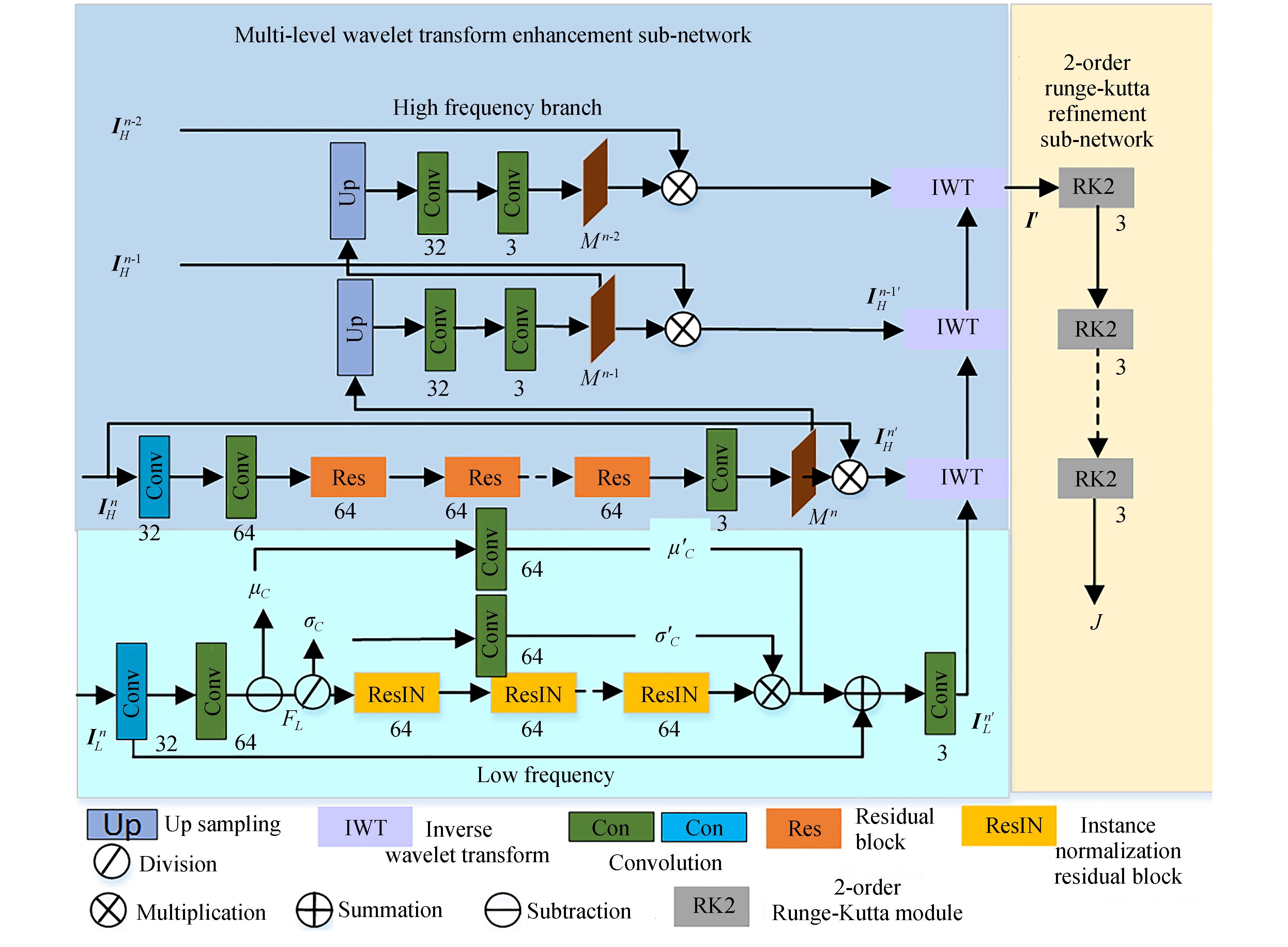
Set citation alerts for the article
Please enter your email address