GAO Fei, LEI Tao, LIU Xianyuan, CHEN Lianghong, JIANG Ping. Super-resolution simplification network based on densely connected structure[J]. Journal of Applied Optics, 2019, 40(5): 805

Search by keywords or author
- Journal of Applied Optics
- Vol. 40, Issue 5, 805 (2019)
Abstract
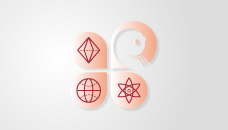
Set citation alerts for the article
Please enter your email address