Mingna ZHANG, Xiaoqi LÜ, Yu GU. Image registration based on residual mixed attention and multi-resolution constraints[J]. Optics and Precision Engineering, 2022, 30(10): 1203

Search by keywords or author
- Optics and Precision Engineering
- Vol. 30, Issue 10, 1203 (2022)
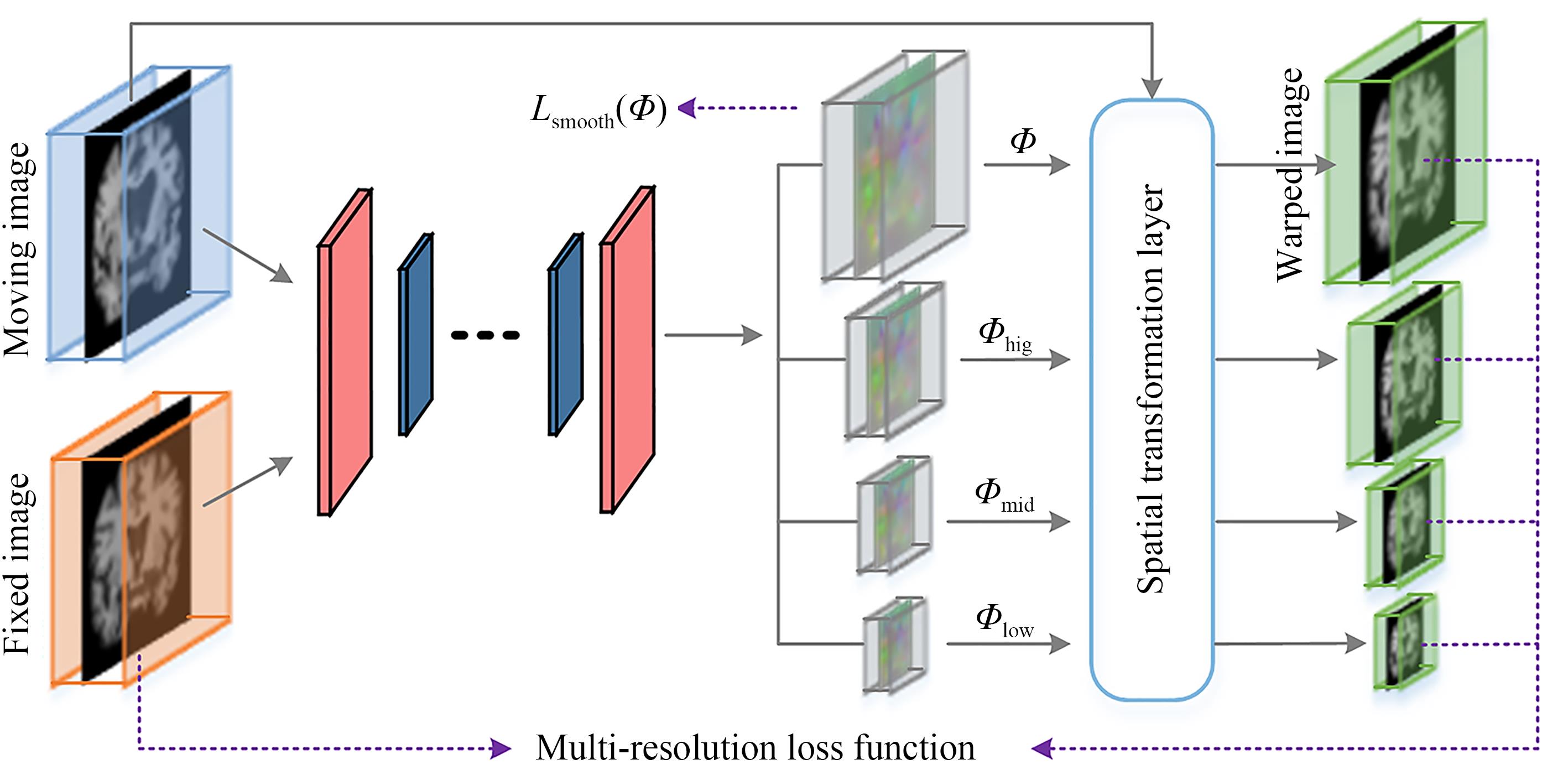
Fig. 1. Diagram of registration network model

Fig. 2. Architecture of MAMReg-Net network
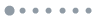
Fig. 3. Diagram of residual mixed attention
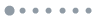
Fig. 4. Structure of Mask branching
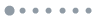
Fig. 5. Non-Local module
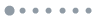
Fig. 6. Images before and after preprocessing
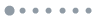
Fig. 7. Image of 12 examples of anatomical structures
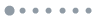
Fig. 8. Samples registration result from test images
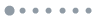
Fig. 9. Color overlay images before and after registration
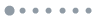
Fig. 10. Registration results using different methods
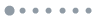
Fig. 11. Histogram of the average Dice score of the 12 anatomical structures on the test images
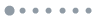
Fig. 12. Histogram of the average ASD score of the 12 anatomical structures on the test images
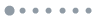
Fig. 13. Dice score boxplot of 12 anatomical structures in ablation experiment
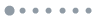
Fig. 14. ASD score boxplot of 12 anatomical structures in ablation experiment
|
Table 1. Registration accuracy of different methods
|
Table 2. Comparison of registration time between different methods
|
Table 3. Comparison of registration accuracy of ABIDE dataset
|
Table 4. Comparison of registration accuracy of ablation experiments
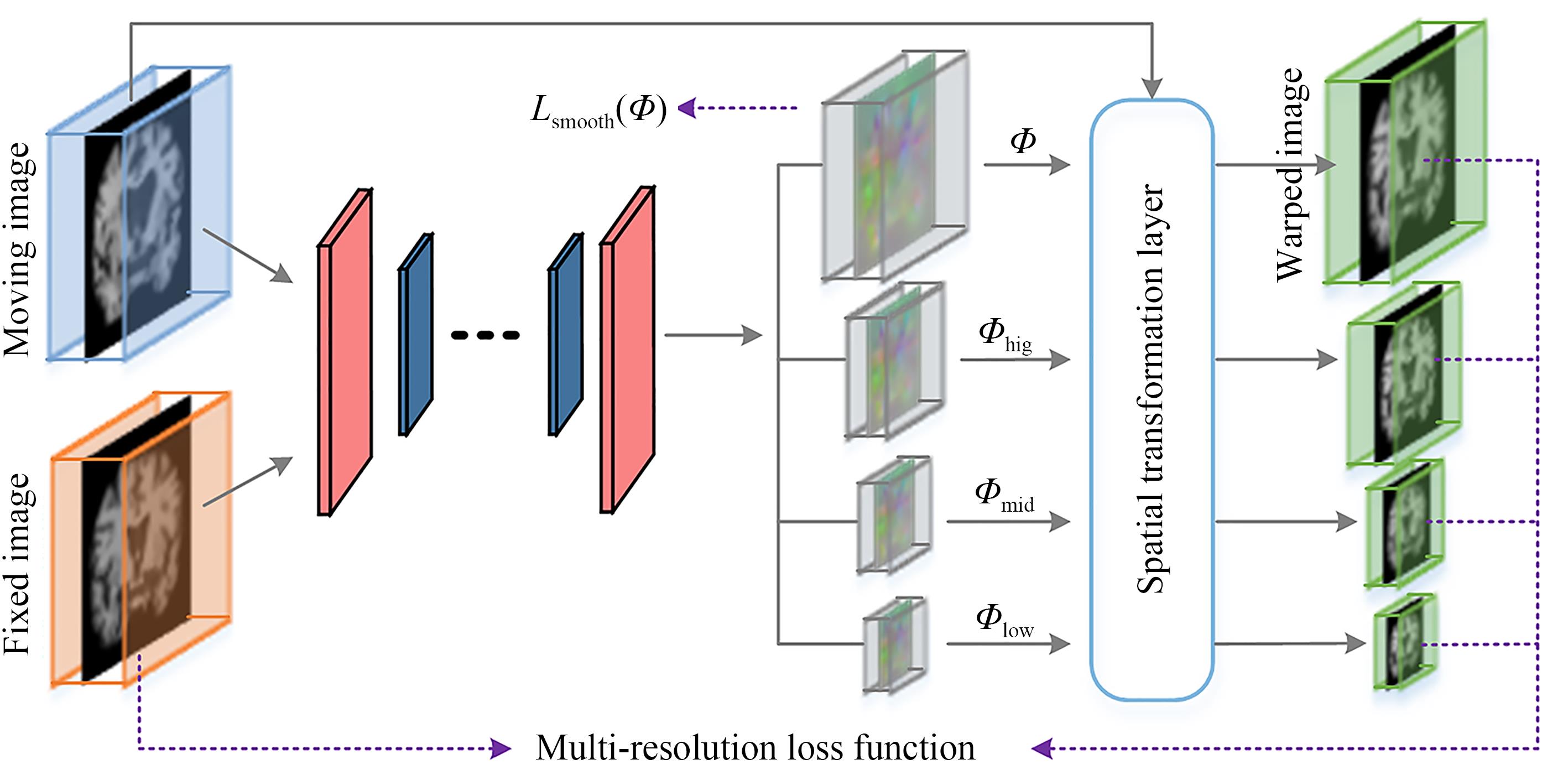
Set citation alerts for the article
Please enter your email address