Anneng Luo, Haibin Wan, Zhiwei Si, Tuanfa Qin. Detection Algorithm of Recyclable Garbage Based on Improved YOLOv5s[J]. Laser & Optoelectronics Progress, 2023, 60(10): 1010010

Search by keywords or author
- Laser & Optoelectronics Progress
- Vol. 60, Issue 10, 1010010 (2023)
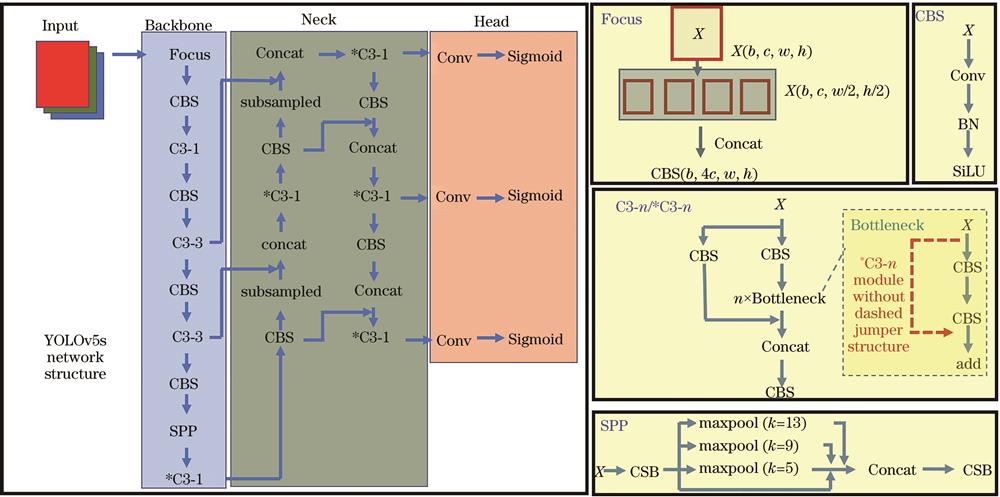
Fig. 1. YOLOv5s network structure

Fig. 2. Basic unit of ShuffleNet v2
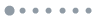
Fig. 3. DW module unit
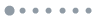
Fig. 4. Structure diagram. (a) Schematic of Neck fusion; (b) schematic of improved YOLOv5s overall network
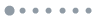
Fig. 5. Statistic on number of labels in each class on the dataset
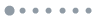
Fig. 6. Original image and image obtained by modules.(a) Original image; (b) image obtained by CBS module; (c) image obtained by S-b module
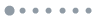
Fig. 7. Comparison of indicators between the improved model and YOLOv5s in training process
|
Table 1. Configuration of backbone network structure and parameters
|
Table 2. Ablation experiment data of general category
|
Table 3. Experimental data for ablation of individual class
|
Table 4. Comparison experiments with common models
|
Table 5. Comparison of processing time at Jeson Nano
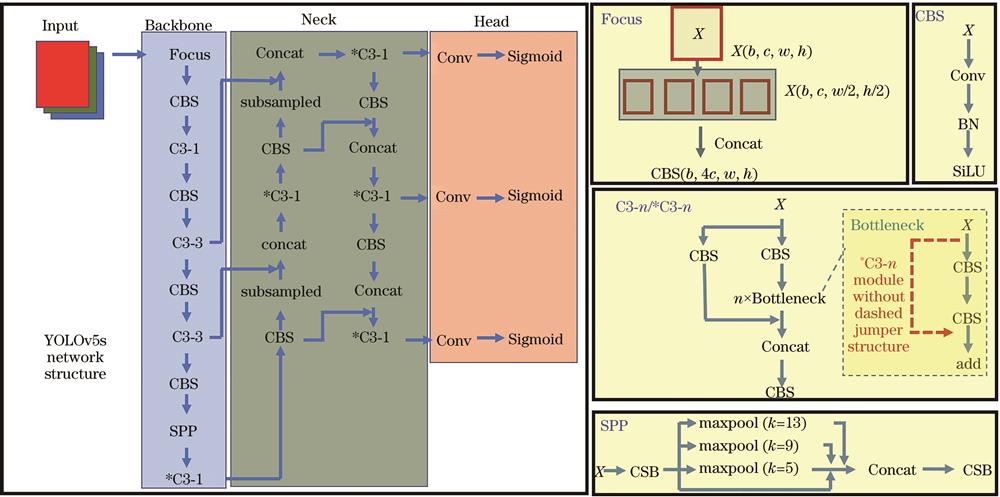
Set citation alerts for the article
Please enter your email address