HUANG Hong, LI Zheng-ying, SHI Guang-yao, PAN Yin-song. Multi-features manifold discriminant embedding for hyperspectral image classification[J]. Optics and Precision Engineering, 2019, 27(3): 726

Search by keywords or author
- Optics and Precision Engineering
- Vol. 27, Issue 3, 726 (2019)
Abstract
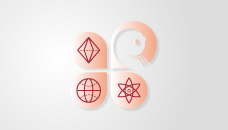
Set citation alerts for the article
Please enter your email address