Linian Ruan, Yan Dong. Non-Subsampling Shearlet Transform Remote Sensing Image Fusion with Improved Dual-channel Adaptive Pulse Coupled Neural Network[J]. Laser & Optoelectronics Progress, 2023, 60(10): 1028004

Search by keywords or author
- Laser & Optoelectronics Progress
- Vol. 60, Issue 10, 1028004 (2023)

Fig. 1. Frequency domain subdivision diagram and support interval of NSST. (a) Frequency domain subdivision map; (b) frequency domain support interval
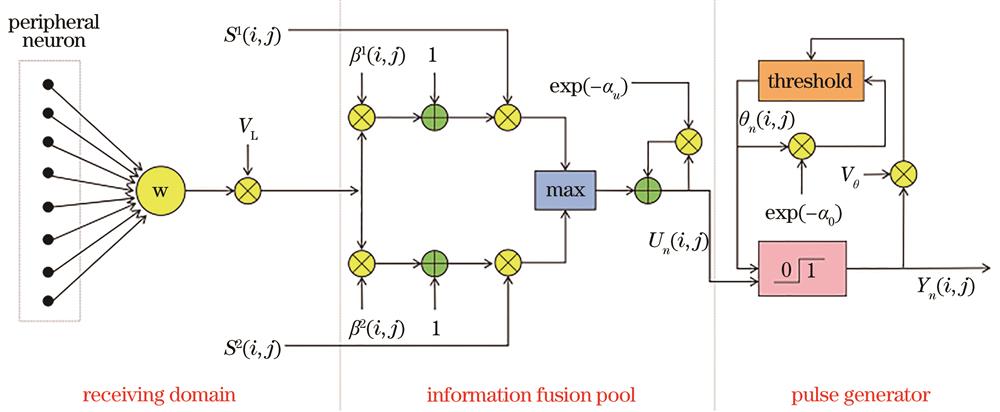
Fig. 2. Architecture of DC-PCNN
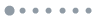
Fig. 3. Fusion results of high-frequency coefficients in all directions. (a)-(b) High frequency subbands in 2 directions in first layer; (c)-(f) high frequency subbands in 4 directions in second layer
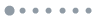
Fig. 4. Direction information calculation
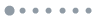
Fig. 5. Flow chart
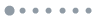
Fig. 6. Influence of decomposition layers on fusion effect
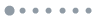
Fig. 7. First group of experimental data. (a) MS; (b) PAN
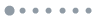
Fig. 8. Fusion results of first group. (a) SE; (b) NSCT; (c) ISCM; (d) WDCPAPCNN; (e) PAPCNN; (f) proposed method
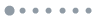
Fig. 9. Second group of experimental data. (a) MS; (b) PAN
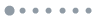
Fig. 10. Fusion results of second group. (a) SE; (b) NSCT; (c) ISCM; (d) WDCPAPCNN; (e) PAPCNN; (f) proposed method
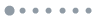
Fig. 11. Third group of experimental data. (a) MS; (b) PAN
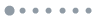
Fig. 12. Fusion results of third group. (a) SE; (b) NSCT; (c) ISCM; (d) WDCPAPCNN; (e) PAPCNN; (f) proposed method
|
Table 1. Quantitative evaluation of spatial information in all directions
|
Table 2. Settings of NSST decomposition layers and corresponding directions
|
Table 3. Quantitative evaluation of first group of experiments
|
Table 4. Quantitative evaluation of second group of experiments
|
Table 5. Quantitative evaluation of third group of experiments

Set citation alerts for the article
Please enter your email address