Dingbang Fang, Gui Feng, Haiyan Cao, Hengjie Yang, Xue Han, Yincheng Yi. Handwritten Formula Symbol Recognition Based on Multi-Feature Convolutional Neural Network[J]. Laser & Optoelectronics Progress, 2019, 56(7): 072001

Search by keywords or author
- Laser & Optoelectronics Progress
- Vol. 56, Issue 7, 072001 (2019)

Fig. 1. Class distribution of 101 symbols

Fig. 2. Images randomly generated after original images passing through elastic distortion model. (a) Original images; (b) images randomly generated for first time; (c) images randomly generated for second time
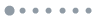
Fig. 3. Structural diagram of DenseNet-SE network
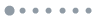
Fig. 4. Structural diagram of residual-dense block module
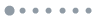
Fig. 5. Validation accuracy comparison of DenseNet and DenseNet-SE
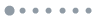
Fig. 6. Accuracy comparison of DenseNet-SE test set and validation set
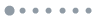
Fig. 7. Symbols of misjudgment types in CROHME2016 test set
|
Table 1. Distribution of CROHME experimental datasets
|
Table 2. Time consumption and accuracy for each epoch test
|
Table 3. Comparison between proposed method and different types of systems
|
Table 4. Symbols of TOP-10 error discrimination types in CROHME2016

Set citation alerts for the article
Please enter your email address