LU Yingying, SUN Xiangyu, JI Weiliang, XING Zhanqiang. Convolutional Neural Network accelerator based on computing in memory[J]. Journal of Terahertz Science and Electronic Information Technology , 2025, 23(2): 170

Search by keywords or author
Journals >Journal of Terahertz Science and Electronic Information Technology >Volume 23 >Issue 2 >Page 170 > Article
- Journal of Terahertz Science and Electronic Information Technology
- Vol. 23, Issue 2, 170 (2025)
Abstract
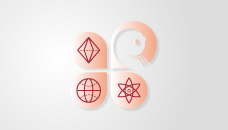
Set citation alerts for the article
Please enter your email address