GU Xuejing, YANG Zhaohui, GUO Yucheng, XU Jingang. EEG Classification Based on Dimensional Attention and Multi-scale Convolutional Networks[J]. Semiconductor Optoelectronics, 2024, 45(1): 152

Search by keywords or author
- Semiconductor Optoelectronics
- Vol. 45, Issue 1, 152 (2024)
Abstract
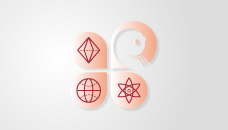
Set citation alerts for the article
Please enter your email address