ZHOU Conghang, LI Jianxing, SHI Yujing, LIN Zhirui, LIN Hanghang. Application of Deep Reinforcement Learning in Path Planning of UAV Formation[J]. Electronics Optics & Control, 2024, 31(10): 27

Search by keywords or author
- Electronics Optics & Control
- Vol. 31, Issue 10, 27 (2024)
Abstract
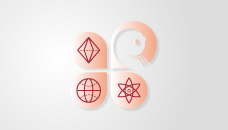
Set citation alerts for the article
Please enter your email address