Lei Xiao, Peng Hu, Junjie Ma. Self-Supervised Monocular Depth Estimation Model Based on Global Information Correlation Under Influence of Local Attention[J]. Laser & Optoelectronics Progress, 2025, 62(8): 0815010

Search by keywords or author
- Laser & Optoelectronics Progress
- Vol. 62, Issue 8, 0815010 (2025)
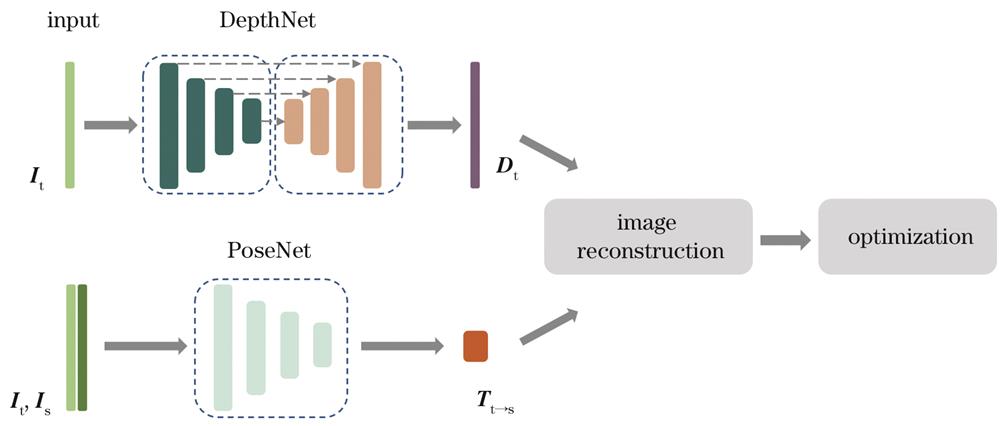
Fig. 1. Overall framework of proposed network
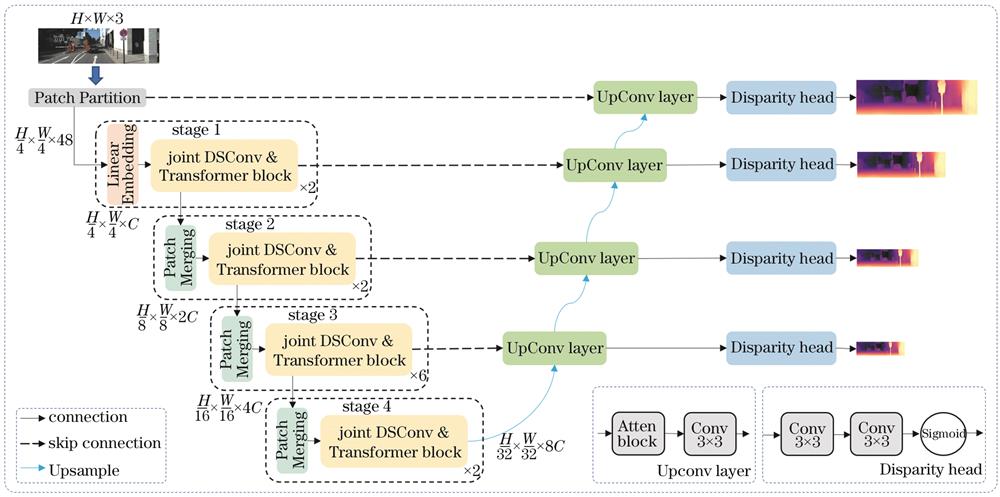
Fig. 2. Internal structure of the DepthNet
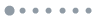
Fig. 3. Schematic diagram of the internal structure for the joint DSConv & Transformer block
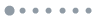
Fig. 4. Qualitative comparison between the proposed method and other methods on the KITTI dataset
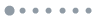
Fig. 5. Qualitative comparison of the proposed method and other methods on the Cityscapes dataset
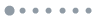
Fig. 6. Model complexity and speed evaluation. (a) Params; (b) FLOPs
|
Table 1. Quantitative comparison between the proposed method and other monocular depth estimation methods on the KITTI dataset
|
Table 2. Quantitative comparison between the proposed method and other monocular depth estimation methods on the Cityscapes dataset
|
Table 3. Ablation experimental results
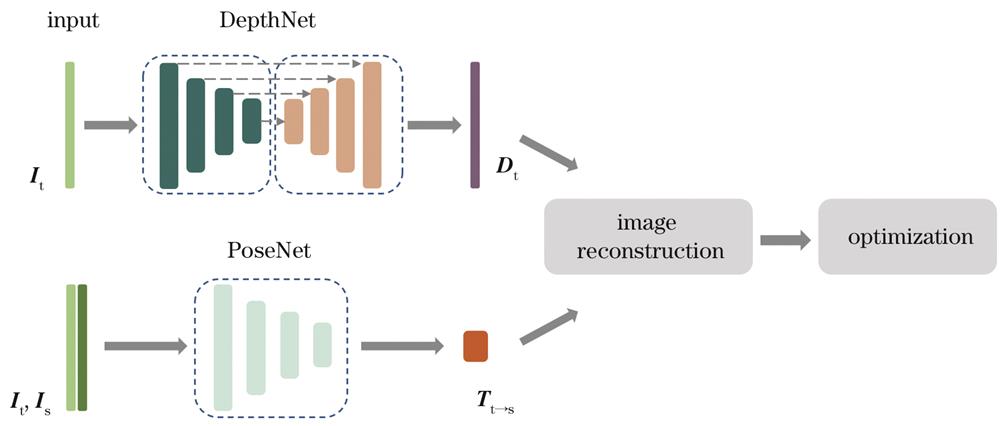
Set citation alerts for the article
Please enter your email address