WANG Xue-mei, YUMITI Maiming, HUANG Xiao-yu, LI Rui, LIU Dong. Estimation of Arsenic Content in Soil Based on Continuous Wavelet Transform[J]. Spectroscopy and Spectral Analysis, 2023, 43(1): 206

Search by keywords or author
- Spectroscopy and Spectral Analysis
- Vol. 43, Issue 1, 206 (2023)
Abstract
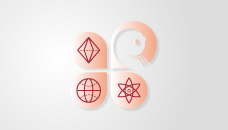
Set citation alerts for the article
Please enter your email address