Fangrui Lin, Yiqiang Wang, Min Yi, Chenshuang Zhang, Liwei Liu, Junle Qu. Research Progress on Fast Fluorescence Lifetime Imaging Microscopy and Its in vivo Applications (Invited)[J]. Laser & Optoelectronics Progress, 2024, 61(6): 0618005

Search by keywords or author
- Laser & Optoelectronics Progress
- Vol. 61, Issue 6, 0618005 (2024)
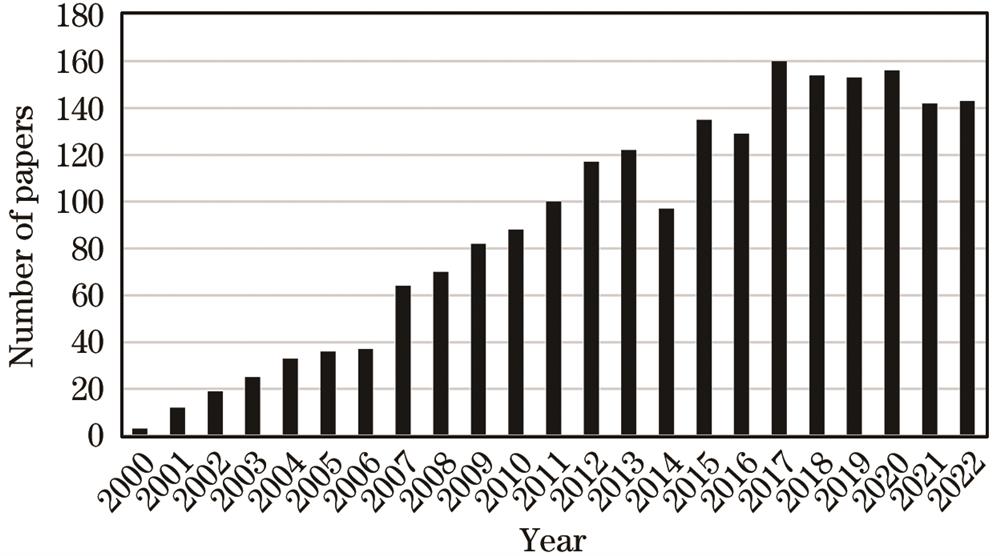
Fig. 1. Number of papers focusing on FLIMfor in vivo imaging
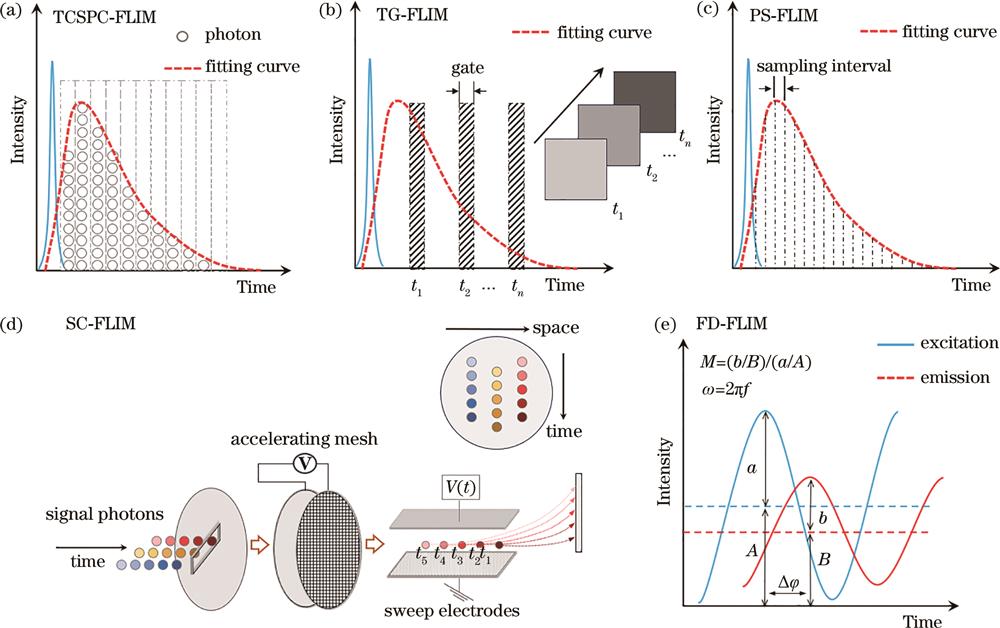
Fig. 2. Principle diagram of TD-FLIM and FD-FLIM
![Imaging speed improving of TCSPC-FLIM by optimizing detection methods. (a) Using a collection module with a shorter dead time to record multiple photons within a single pulse cycle[36]; (b) using a photon'Spinner' to shorten the dead time of the capture card[37]; (c) using parallel array detection modules to improve photon counting rate[38]](/Images/icon/loading.gif)
Fig. 3. Imaging speed improving of TCSPC-FLIM by optimizing detection methods. (a) Using a collection module with a shorter dead time to record multiple photons within a single pulse cycle[36]; (b) using a photon'Spinner' to shorten the dead time of the capture card[37]; (c) using parallel array detection modules to improve photon counting rate[38]
![Imaging speed improving of TCSPC-FLIM by optimizing scanning methods. (a) Implementing fast scanning with arbitrary shapes based on 2D-AOD[39]; (b) using a multifocal array to achieve fast scanning[41]; (c) using line excitation to realize fast scanning, and the imaging results of two slices shown on right[44]](/Images/icon/loading.gif)
Fig. 4. Imaging speed improving of TCSPC-FLIM by optimizing scanning methods. (a) Implementing fast scanning with arbitrary shapes based on 2D-AOD[39]; (b) using a multifocal array to achieve fast scanning[41]; (c) using line excitation to realize fast scanning, and the imaging results of two slices shown on right[44]
![Schematic of TG-FLIM system based on light-sheet[55]. (a) Schematic of the system; (b) imaging results of a living zebrafish](/Images/icon/loading.gif)
Fig. 5. Schematic of TG-FLIM system based on light-sheet[55]. (a) Schematic of the system; (b) imaging results of a living zebrafish
![SC-FLIM technique for rapid imaging. (a) Single particle tracking FLIM based on double helix point spread function engineering[67]; (b) implementation of high-speed FLIM using compressed sensing-based DMD spatial encoding technology[68]](/Images/icon/loading.gif)
Fig. 6. SC-FLIM technique for rapid imaging. (a) Single particle tracking FLIM based on double helix point spread function engineering[67]; (b) implementation of high-speed FLIM using compressed sensing-based DMD spatial encoding technology[68]
![Widefield FD-FLIM based on modulation of LED[70]. (a) Schematic of the system; (b) fluorescence intensity images of Fluorescein-Glycerol (Fl-Gly) solutions with different ratios; (c) corresponding fluorescence lifetime images](/Images/icon/loading.gif)
Fig. 7. Widefield FD-FLIM based on modulation of LED[70]. (a) Schematic of the system; (b) fluorescence intensity images of Fluorescein-Glycerol (Fl-Gly) solutions with different ratios; (c) corresponding fluorescence lifetime images
![Correcting motion artifacts for in vivo FLIM through post-processing. (a) Inter frame artifact correction based on normalized cross-correlation algorithm[77]; (b) inter frame and intra frame artifact correction based on the Lucas-Kanade framework[78]](/Images/icon/loading.gif)
Fig. 8. Correcting motion artifacts for in vivo FLIM through post-processing. (a) Inter frame artifact correction based on normalized cross-correlation algorithm[77]; (b) inter frame and intra frame artifact correction based on the Lucas-Kanade framework[78]
![Flowchart of ADCG algorithm and the fitting results with photon number of 45[86]](/Images/icon/loading.gif)
Fig. 9. Flowchart of ADCG algorithm and the fitting results with photon number of 45[86]
![Three types of DL algorithms contribute to reduce FLIM processing time. (a) Traditional curve fitting is substituted by either a 3D-CNN[92] (left) or a 1D-CNN[93] (right) framework; (b) LLE and NIII sub networks are utilized to produce data with high photon counts from low photon counts[96]; (c) DL algorithms are employed to improve imaging resolution[97]](/Images/icon/loading.gif)
Fig. 10. Three types of DL algorithms contribute to reduce FLIM processing time. (a) Traditional curve fitting is substituted by either a 3D-CNN[92] (left) or a 1D-CNN[93] (right) framework; (b) LLE and NIII sub networks are utilized to produce data with high photon counts from low photon counts[96]; (c) DL algorithms are employed to improve imaging resolution[97]
![Schematic of instant FLIM technique and its in vivo imaging results of neurons in mouse brain[71]](/Images/icon/loading.gif)
Fig. 11. Schematic of instant FLIM technique and its in vivo imaging results of neurons in mouse brain[71]
![TCSPC-FLIM for living mice. (a) Imaging brain vessels and Aβ plaque in AD mice[103]; (b) quantitative characterization of intracellular glucose concentration in cortical neurons[104]; (c) three photon FLIM of cerebral vessels[105]; (d) imaging of different organs in mice based on microendoscopy[106]](/Images/icon/loading.gif)
Fig. 12. TCSPC-FLIM for living mice. (a) Imaging brain vessels and Aβ plaque in AD mice[103]; (b) quantitative characterization of intracellular glucose concentration in cortical neurons[104]; (c) three photon FLIM of cerebral vessels[105]; (d) imaging of different organs in mice based on microendoscopy[106]
![Applications of FLIM for intraoperative tumor diagnosis. (a) Recognition of tumor boundary based on PS-FLIM[108]; (b) high-resolution recognition of tumor cells based on TCSPC-FLIM[115]; (c) fast FD-FLIM with a large field-of-view based on 5-ALA labeling[117]](/Images/icon/loading.gif)
Fig. 13. Applications of FLIM for intraoperative tumor diagnosis. (a) Recognition of tumor boundary based on PS-FLIM[108]; (b) high-resolution recognition of tumor cells based on TCSPC-FLIM[115]; (c) fast FD-FLIM with a large field-of-view based on 5-ALA labeling[117]
|
Table 1. Performance comparison of several fast FLIM technologies based on DL
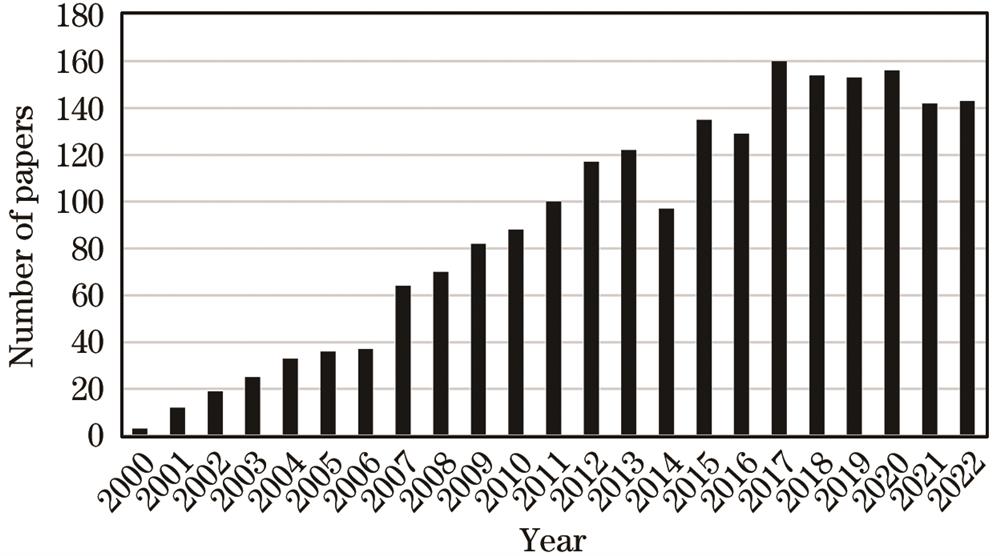
Set citation alerts for the article
Please enter your email address