HOU Zhouyang, YANG Liqiong, ZHANG Guiying, XIAO Yufeng. Deep Learning-Based Apparent Defect Detection in Bridges[J]. Semiconductor Optoelectronics, 2024, 45(6): 990

Search by keywords or author
- Semiconductor Optoelectronics
- Vol. 45, Issue 6, 990 (2024)
Abstract
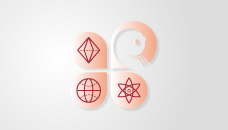
Set citation alerts for the article
Please enter your email address