Zihan Xiong, Liangfeng Song, Xin Liu, Chao Zuo, Peng Gao. Performance enhancement of fluorescence microscopy by using deep learning (invited)[J]. Infrared and Laser Engineering, 2022, 51(11): 20220536

Search by keywords or author
- Infrared and Laser Engineering
- Vol. 51, Issue 11, 20220536 (2022)
![Diagram of the structure of a commonly used neural network. (a) Convolutional neural network; (b) U-Net architecture[31]; (c) Recurrent neural network; (d) Generative adversarial networks[58]](/richHtml/irla/2022/51/11/20220536/img_1.jpg)
Fig. 1. Diagram of the structure of a commonly used neural network. (a) Convolutional neural network; (b) U-Net architecture[31]; (c) Recurrent neural network; (d) Generative adversarial networks[58]
![Deep learning network for spatial resolution enhancement of wide-field imaging and confocal microscopy[25]. (a) Architecture of generative adversarial network (GAN) used for image super-resolution; (b1)-(b3) Imaging results of bovine pulmonary artery endothelial cells (BPAECs), (b1) Network input image acquired with a 10×/0.4 NA objective lens, (b2) Network output image, (b3) Image acquired with a 20×/0.75 NA objective lens; (c1) Confocal images of fluorescent spheres; (c2) Cross-modality images obtained using the network; (c3) Super-resolution images obtained by STED](/richHtml/irla/2022/51/11/20220536/img_2.jpg)
Fig. 2. Deep learning network for spatial resolution enhancement of wide-field imaging and confocal microscopy[25]. (a) Architecture of generative adversarial network (GAN) used for image super-resolution; (b1)-(b3) Imaging results of bovine pulmonary artery endothelial cells (BPAECs), (b1) Network input image acquired with a 10×/0.4 NA objective lens, (b2) Network output image, (b3) Image acquired with a 20×/0.75 NA objective lens; (c1) Confocal images of fluorescent spheres; (c2) Cross-modality images obtained using the network; (c3) Super-resolution images obtained by STED
![Super-resolution SIM imaging with U-Net[31]. (a) Fifteen or three SIM raw data images were used as input and the corresponding SIM reconstructions from 15 images were used as the ground truth to train the U-Net; (b) Reconstruction results for different subcellular structures; (c) Intensity distribution of Figure (b) along the dashed line in each image. In the plot, the average is shown on the right y-axis and all others share is the left y-axis](/Images/icon/loading.gif)
Fig. 3. Super-resolution SIM imaging with U-Net[31]. (a) Fifteen or three SIM raw data images were used as input and the corresponding SIM reconstructions from 15 images were used as the ground truth to train the U-Net; (b) Reconstruction results for different subcellular structures; (c) Intensity distribution of Figure (b) along the dashed line in each image. In the plot, the average is shown on the right y -axis and all others share is the left y -axis
![Deep learning for resolution enhancement of light-field micro-scopy [37]. (a) Imaging of RBCs in the beating zebrafish heart using VCD-LFM and LFDM; (b) Imaging of cardiomyocyte nuclei in the beating zebrafish heart using VCD- LFM and LFDM](/Images/icon/loading.gif)
Fig. 4. Deep learning for resolution enhancement of light-field micro-scopy [37]. (a) Imaging of RBCs in the beating zebrafish heart using VCD-LFM and LFDM; (b) Imaging of cardiomyocyte nuclei in the beating zebrafish heart using VCD- LFM and LFDM
![Volumetric imaging through Recurrent-MZ[40]. (a) Recurrent-MZ volumetric imaging framework.M is the number of input scans (2D images), and each input scan is paired with its corresponding DPM (Digital Propagation Matrix); (b) Recurrent-MZ network structure](/Images/icon/loading.gif)
Fig. 5. Volumetric imaging through Recurrent-MZ[40]. (a) Recurrent-MZ volumetric imaging framework.M is the number of input scans (2D images), and each input scan is paired with its corresponding DPM (Digital Propagation Matrix); (b) Recurrent-MZ network structure
![DSP-Net for 3D imaging of mouse brain[44]. (a) Work flow of DSP-Net; (b) DSP-Net–enabled LSFM results of the fluorescence-labelled neurons in the mouse brain](/Images/icon/loading.gif)
Fig. 6. DSP-Net for 3D imaging of mouse brain[44]. (a) Work flow of DSP-Net; (b) DSP-Net–enabled LSFM results of the fluorescence-labelled neurons in the mouse brain
![Fluorescence microscopy image datasets with different noise levels. The single-channel (gray) images are acquired with two-photon microscopy on fixed mouse brain tissues. The multi-channel (color) images are obtained with two-photon microscopy on fixed BPAE cells. The ground truth images are estimated by averaging 50 noisy raw images[91]](/Images/icon/loading.gif)
Fig. 7. Fluorescence microscopy image datasets with different noise levels. The single-channel (gray) images are acquired with two-photon microscopy on fixed mouse brain tissues. The multi-channel (color) images are obtained with two-photon microscopy on fixed BPAE cells. The ground truth images are estimated by averaging 50 noisy raw images[91]
![Architecture of the SRACNet for fluorescence micrograph enhancement[45]. (a) Training processes of SRACNet architecture. The numbers inside the circle arrows represent the repeat times of the residual blocks. The numbers under each level represent the number of channels. The subscript i represents different scales; (b) Schematic diagram of the modified residual block architecture. “N” and “N/2” represent the number of channels](/Images/icon/loading.gif)
Fig. 8. Architecture of the SRACNet for fluorescence micrograph enhancement[45]. (a) Training processes of SRACNet architecture. The numbers inside the circle arrows represent the repeat times of the residual blocks. The numbers under each level represent the number of channels. The subscript i represents different scales; (b) Schematic diagram of the modified residual block architecture. “N ” and “N /2” represent the number of channels
![ScatNet restoration of neuronal signals in mouse brain slices (Thy1-YFP-M) imaged by TPEM[46]. (a) The raw imaging results through 0 µm (ground-truth) brain slices; (b) Left: the raw imaging results through 100 µm brain slices, Right: the results restored from the scattered signals in Left; (c) Left: the raw imaging results through 200 µm brain slices, Right: the results restored from the scattered signals in Left; (d) Left: the raw imaging results through 300 µm brain slices, Right: the results restored from the scattered signals in Left](/Images/icon/loading.gif)
Fig. 9. ScatNet restoration of neuronal signals in mouse brain slices (Thy1-YFP-M) imaged by TPEM[46]. (a) The raw imaging results through 0 µm (ground-truth) brain slices; (b) Left: the raw imaging results through 100 µm brain slices, Right: the results restored from the scattered signals in Left; (c) Left: the raw imaging results through 200 µm brain slices, Right: the results restored from the scattered signals in Left; (d) Left: the raw imaging results through 300 µm brain slices, Right: the results restored from the scattered signals in Left
![Deep learning-based implementation of virtual fluorescent labeling[94]. (a) Deep neural network train process for predicting fluorescent markers from unlabeled images; (b)-(d) Transmitted light images of the same cells, fluorescent images, and fluorescent markers predicted with the ISL model](/Images/icon/loading.gif)
Fig. 10. Deep learning-based implementation of virtual fluorescent labeling[94]. (a) Deep neural network train process for predicting fluorescent markers from unlabeled images; (b)-(d) Transmitted light images of the same cells, fluorescent images, and fluorescent markers predicted with the ISL model
![Unsupervised image transformation network framework for virtual fluorescence imaging[97]. (a) Autofluorescence image of unlabeled tissue cores; (b) H&E staining predicted by UTOM; (c) Bright-field image of the corresponding adjacent H&E-stained section for comparison](/Images/icon/loading.gif)
Fig. 11. Unsupervised image transformation network framework for virtual fluorescence imaging[97]. (a) Autofluorescence image of unlabeled tissue cores; (b) H&E staining predicted by UTOM; (c) Bright-field image of the corresponding adjacent H&E-stained section for comparison
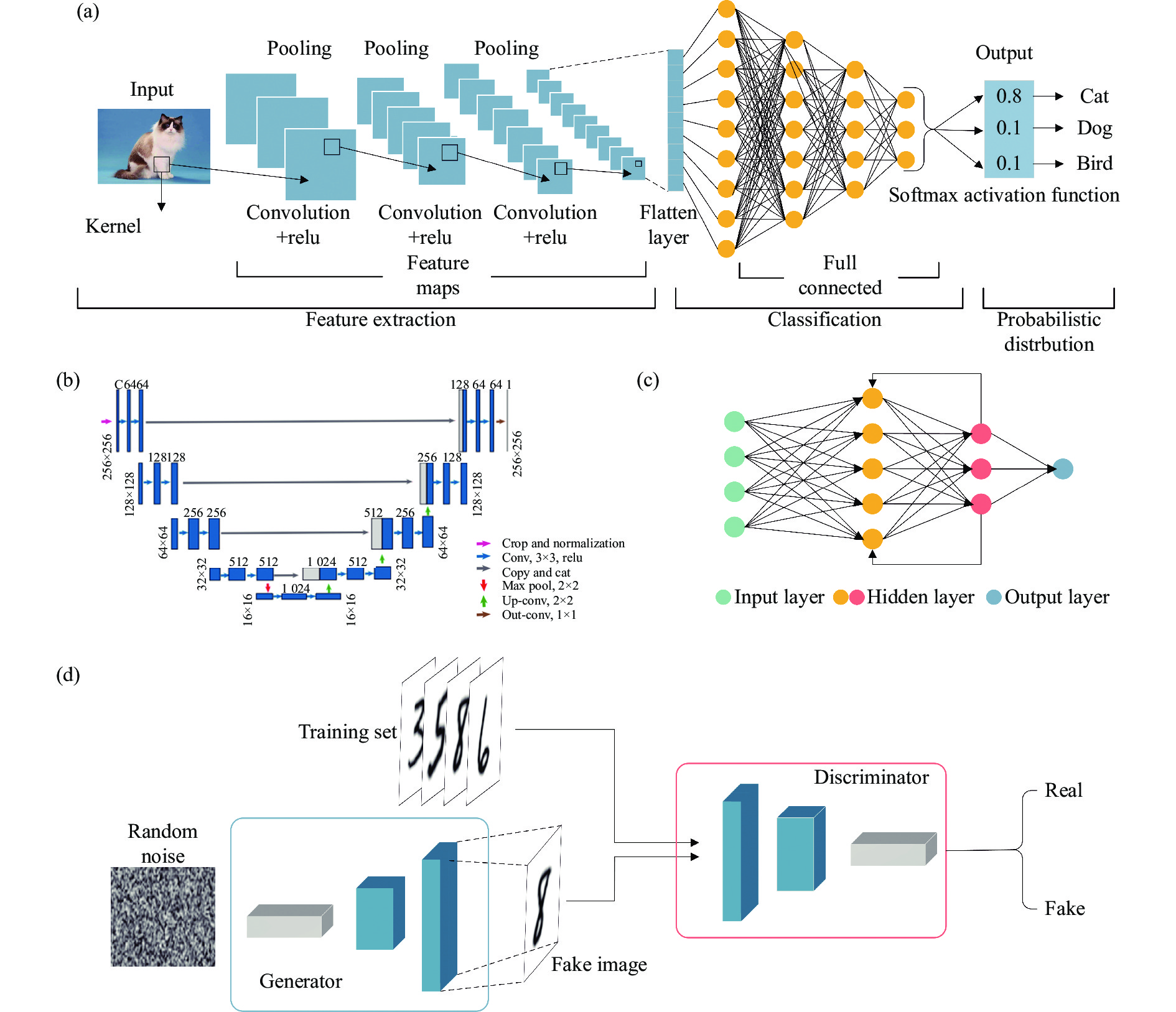
Set citation alerts for the article
Please enter your email address