Xi Gong, Zhanlong Chen, Liang Wu, Zhong Xie, Yongyang Xu. Transfer Learning Based Mixture of Experts Classification Model for High-Resolution Remote Sensing Scene Classification[J]. Acta Optica Sinica, 2021, 41(23): 2301003

Search by keywords or author
- Acta Optica Sinica
- Vol. 41, Issue 23, 2301003 (2021)

Fig. 1. Flow chart of TLMoE

Fig. 2. Transfer learning process of expert network
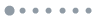
Fig. 3. Training sample filter for expert networks
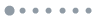
Fig. 4. Image examples of remote sensing scenes. (a) UCM dataset; (b) SIRI dataset; (c) RSSCN7 dataset
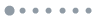
Fig. 5. Classification confusion matrix of TLMoE-VGG19 on UCM dataset
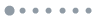
Fig. 6. Classification confusion matrix of TLMoE-VGG19 on SIRI dataset
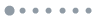
Fig. 7. Classification confusion matrix of TLMoE-VGG19 on RSSCN7 dataset
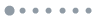
Fig. 8. Time consumption comparison before and after the combination of channels and pre-trained CNN in TLMoE. (a) VGG19; (b) Resnet50
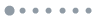
Fig. 9. Comparison of different features on the 3 datasets by 2-dimensional feature visualization
|
Table 1. Structure comparison between VGG19 and Resnet50
|
Table 2. Classification accuracy comparison on the UCM dataset
|
Table 3. Classification accuracy comparison on the confusing classes of UCM datasetunit: %
|
Table 4. Classification accuracy comparison on the SIRI dataset
|
Table 5. Classification accuracy comparison on the RSSCN7 dataset
|
Table 6. Classification accuracy comparison between TLMoE channels
|
Table 7. Classification accuracy comparison of several kinds of features

Set citation alerts for the article
Please enter your email address