Yiduo Li, Zibo Guo, Kai Liu, Xiaoyao Sun. Mixed-precision quantization for neural networks based on error limit (Invited)[J]. Infrared and Laser Engineering, 2022, 51(4): 20220166

Search by keywords or author
- Infrared and Laser Engineering
- Vol. 51, Issue 4, 20220166 (2022)
![(a) Photograph of deep learning convolutional 8-bit quantization procession[6]; (b) The distribution trend of the most valued weights in the first 20 layers of the YOLOV5 s network; (c) Distribution of activation maximum and cutoff value during network quantization in YOLOV5 s](/richHtml/irla/2022/51/4/20220166/img_1.jpg)
Fig. 1. (a) Photograph of deep learning convolutional 8-bit quantization procession[6]; (b) The distribution trend of the most valued weights in the first 20 layers of the YOLOV5 s network; (c) Distribution of activation maximum and cutoff value during network quantization in YOLOV5 s

Fig. 2. Framework of network hierarchical policy methodology
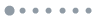
Fig. 3. Example of COCO dataset detection results
|
Table 1. Product quantization method and shift quantization method
|
Table 2. The performance of different quantification methods on the VOC2007 dataset
|
Table 3. Network accuracy before and after quantization with different truncation methods
|
Table 4. Error limit parameter γ value comparison
|
Table 5. Test results of different quantification methods on COCO dataset and VOC2011 dataset
|
Table 6. VOC2011 dataset category accuracy detection table

Set citation alerts for the article
Please enter your email address