Yike Zou, Ying Wu, Jingwen Ma, Yuanyuan Huang, Jinghui Ning, Qijia Fu. Neural Network Inversion Method for Atmospheric Temperature and Relative Humidity Profiles Based on FY-3E/HIRAS[J]. Laser & Optoelectronics Progress, 2025, 62(7): 0701001

Search by keywords or author
- Laser & Optoelectronics Progress
- Vol. 62, Issue 7, 0701001 (2025)
Note: This section is automatically generated by AI . The website and platform operators shall not be liable for any commercial or legal consequences arising from your use of AI generated content on this website. Please be aware of this.
Abstract
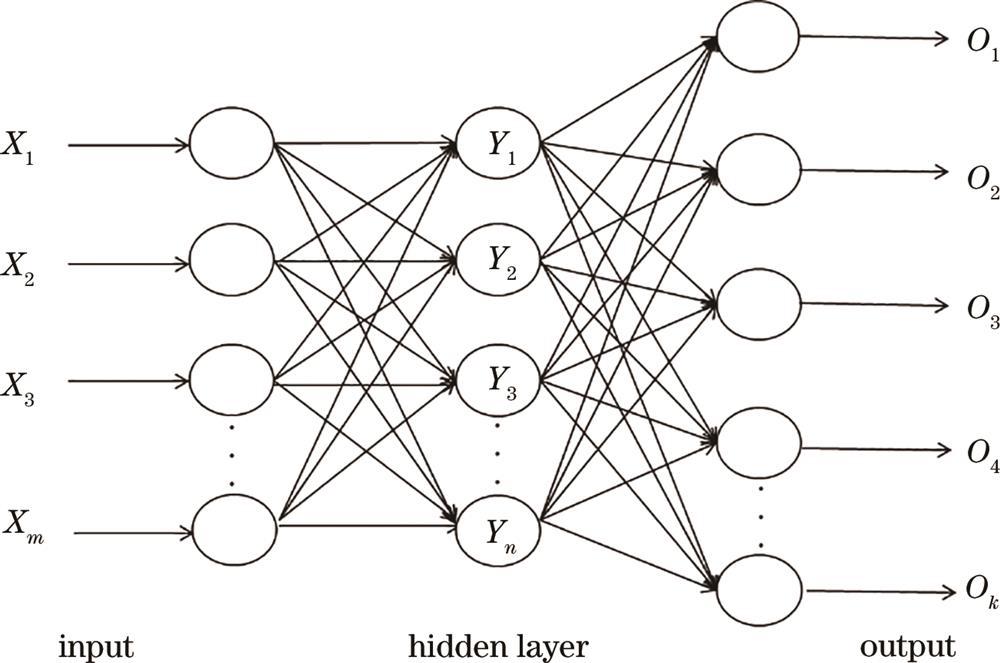
Set citation alerts for the article
Please enter your email address