Yike Zou, Ying Wu, Jingwen Ma, Yuanyuan Huang, Jinghui Ning, Qijia Fu. Neural Network Inversion Method for Atmospheric Temperature and Relative Humidity Profiles Based on FY-3E/HIRAS[J]. Laser & Optoelectronics Progress, 2025, 62(7): 0701001

Search by keywords or author
- Laser & Optoelectronics Progress
- Vol. 62, Issue 7, 0701001 (2025)
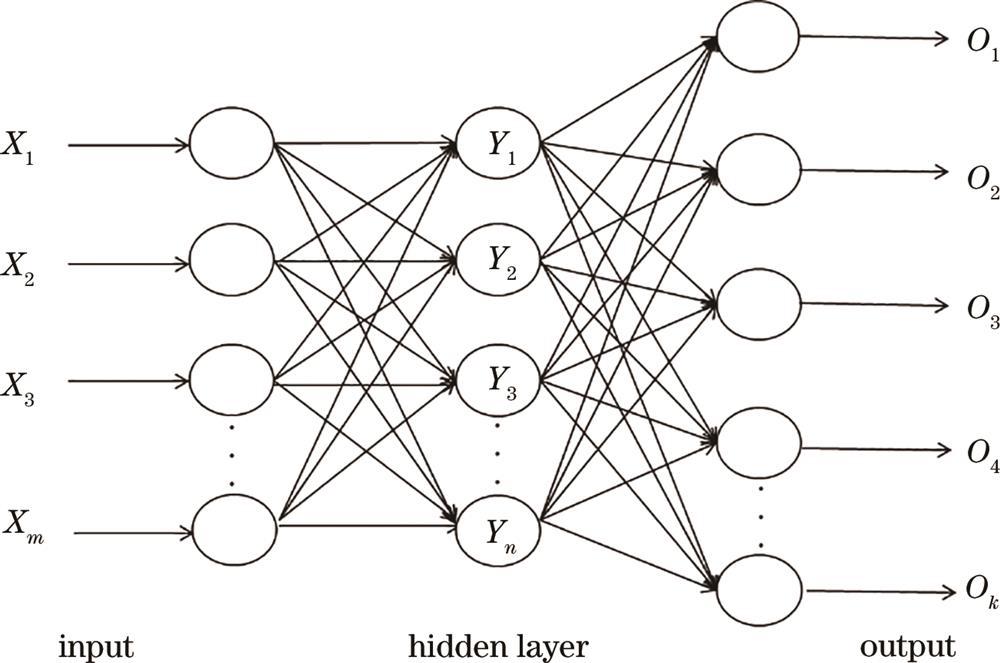
Fig. 1. Structure of three-layer neural network model
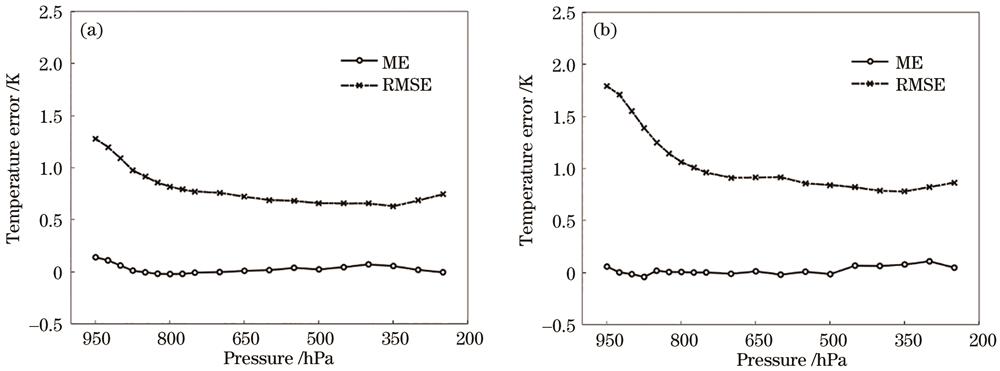
Fig. 2. Variation in temperature inversion error with height on the training set. (a) Clear sky; (b) cloudy sky
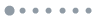
Fig. 3. Variation in temperature inversion error with height on the validation set. (a) Clear sky; (b) cloudy sky
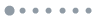
Fig. 4. Variation in RMSE of temperature inversion results with height on the training set. (a) Clear sky; (b) cloudy sky
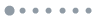
Fig. 5. Variation in RMSE of temperature inversion results with height on the validation set. (a) Clear sky; (b) cloudy sky
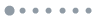
Fig. 6. Variation in relative humidity inversion error with height on the training set. (a) Clear sky; (b) cloudy sky
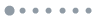
Fig. 7. Variation in relative humidity inversion error with height on the validation set. (a) Clear sky; (b) cloudy sky
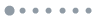
Fig. 8. Variation in RMSE of relative humidity inversion with height in the training set. (a) Clear sky; (b) cloudy sky
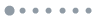
Fig. 9. Variation in RMSE of relative humidity inversion with height in the validation set. (a) Clear sky; (b) cloudy sky
|
Table 1. Parameters of BPNN inversion model
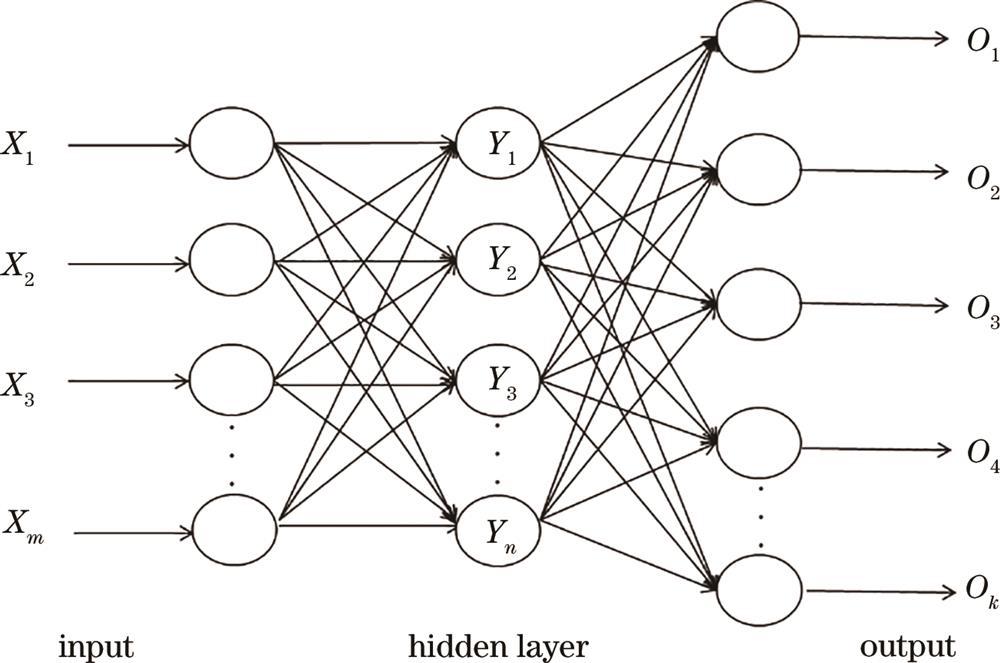
Set citation alerts for the article
Please enter your email address