JIANG Yu-heng, YAN Bo, ZHUANG Qing-yuan, WANG Ai-ping, CAO Shuang, TIAN An-hong, FU Cheng-biao. Quantitative Inversion Model of Soil Heavy Metals Zn and Ni Based on Fractional Order Derivative[J]. Spectroscopy and Spectral Analysis, 2024, 44(10): 2850

Search by keywords or author
- Spectroscopy and Spectral Analysis
- Vol. 44, Issue 10, 2850 (2024)
Abstract
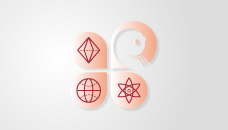
Set citation alerts for the article
Please enter your email address