LIU Yu-juan, LIU Yan-da, YAN Zhen, ZHANG Zhi-yong, CAO Yi-ming, SONG Ying. Classification of Hybrid Convolution Hyperspectral Images Based on Attention Mechanism[J]. Spectroscopy and Spectral Analysis, 2024, 44(10): 2916

Search by keywords or author
- Spectroscopy and Spectral Analysis
- Vol. 44, Issue 10, 2916 (2024)
Abstract
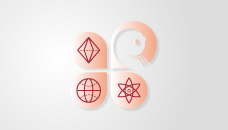
Set citation alerts for the article
Please enter your email address