Sitong Wu, Zhichao Yang, Chenguang Ma, Xun Zhang, Chao Mi, Jiajia Zhou, Zhiyong Guo, Dayong Jin. Deep learning enhanced NIR-II volumetric imaging of whole mice vasculature[J]. Opto-Electronic Advances, 2023, 6(4): 220105

Search by keywords or author
- Opto-Electronic Advances
- Vol. 6, Issue 4, 220105 (2023)

Fig. 1. Trade-offs among lateral resolution, depth resolution, speed, and volume of view by using the three light illumination strategies, wide-field11, spot scanning12, and light-sheet scanning13, in NIR-II fluorescent volumetric imaging (log scale).

Fig. 2. Adjustable large-FoV light-sheet illumination system for high-contrast whole mice imaging. (a ) light-sheet illumination system with adjustable thickness from 183.1 to 100.5 μm and Rayleigh length from 8 to 26 mm, formed by a 30 mm focus lens (Thorlab-1700-B), a 16 mm focus 0.79 NA aspherical lens (Thorlab-ACL254U-B), and a 10 × 0.3 NA objective lens. (b ) The illumination of light sheet through the whole mice. (c ) Comparison of a wide-field imaging result and a top view of light-sheet scanning result at a distance of 100 μm from the mice. (d ) Line profile analysis of (c).
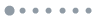
Fig. 3. Phantom experiment to evaluate the scattering effect under the large-FoV light-sheet illumination. (a ) Schematic illustration of light-sheet scanning across the phantom matrix. (b ) Scattering simulation using silicone phantom containing lanthanide doped NIR-II fluorescent nanoparticles and TiO2 nanoparticles. (c ) Letter patterns stained by lanthanide-doped NIR-II fluorescent nanoparticles and embedded in silicone phantom. (d) Reconstruction of the letter patterns by light-sheet scanning. The diameter of the container is 5.5 cm. The step of light-sheet scanning between each layer is 1 mm.
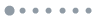
Fig. 4. Time-gated light-sheet imaging system. (a ) TG imaging system. (b ) Comparison results of TG light-sheet imaging and CW filter-based light-sheet imaging with different average laser power. (c ) Icontrast of TG mode and CW mode with different average laser power. (d ) Range of intensity of TG mode and CW mode with different average laser power.
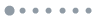
Fig. 5. Comparison schematics and results of vessel enhancement processes by using Hessian matrix v.s. DenseNet deep learning algorithm based on protuberance detection. (a ) Raw image. (b ) Hessian matrix enhancement. (c ) DenseNet enhancement result. (d ) Intensity discriminator. (e –h ) are the profiles of white dotted lines in (a–d). (i ) Training process of Hessian algorithm and our algorithm.
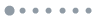
Fig. 6. The depth information of the high contrast images of the whole mice skin and blood vessel revealed by the time-gated light-sheet NIR-II volumetric imaging system enhanced by deep learning algorithm. (a ) The 3D reconstruction image of mice skin and vessel networks. (b ) Luminescent intensity map. (c ) The height map of the mice’s blood vessels. (d ) The height map of the mice’s skin. (e ) Vessels with colors that represent their depth under the skin. (f , g ) Local details of the same position in (a) and (c). (h , i ) Intensity profiles of vessels in different depths. (h) A magnified part of (b). Intensity profiles of lines in (i) with depths of 500 μm, 750 μm, and 1000 μm.

Set citation alerts for the article
Please enter your email address