NI Jin, SUO Li-min, LIU Hai-long, ZHAO Rui. Identification of Corn Varieties Based on Northern Goshawk Optimization Kernel Based Extreme Learning Machine[J]. Spectroscopy and Spectral Analysis, 2024, 44(6): 1584

Search by keywords or author
- Spectroscopy and Spectral Analysis
- Vol. 44, Issue 6, 1584 (2024)
Abstract
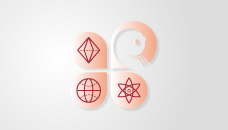
Set citation alerts for the article
Please enter your email address