DAI Yu-jia, GAO Xun, LIU Zi-yuan. Accuracy Improvement of Mn Element in Aluminum Alloy by the Combination of LASSO-LSSVM and Laser-Induced Breakdown Spectroscopy[J]. Spectroscopy and Spectral Analysis, 2024, 44(4): 977

Search by keywords or author
- Spectroscopy and Spectral Analysis
- Vol. 44, Issue 4, 977 (2024)
Abstract
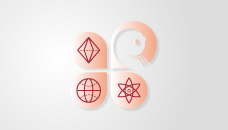
Set citation alerts for the article
Please enter your email address