Qiang Li, Xiyuan Wang, Jiawei He. Improved Algorithm for Super-Resolution Reconstruction of Remote-Sensing Images Based on Generative Adversarial Networks[J]. Laser & Optoelectronics Progress, 2023, 60(10): 1028010

Search by keywords or author
- Laser & Optoelectronics Progress
- Vol. 60, Issue 10, 1028010 (2023)

Fig. 1. Improved SR reconstruction network. (a) Structure of generating network; (b) structure of adversarial network
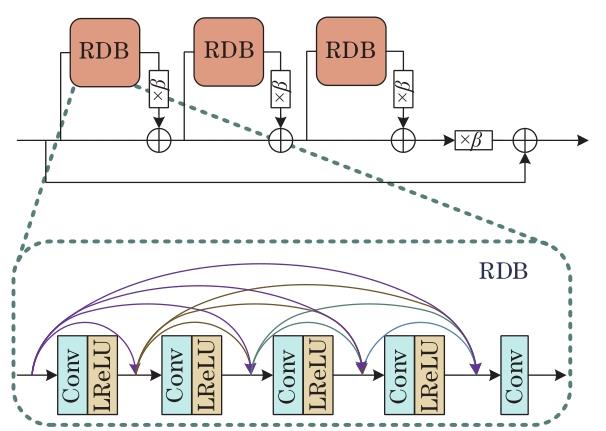
Fig. 2. Structure of RRDB
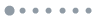
Fig. 3. Structure of RRFDB
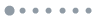
Fig. 4. Structure of RFB
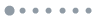
Fig. 5. PSNR values of different convolution combinations
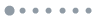
Fig. 6. Image reconstruction of different algorithms on Kaggle test dataset. (a) HR; (b) Bicubic; (c) SRGAN; (d) EDSR; (e) ESRGAN; (f) proposed algorithm
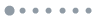
Fig. 7. Image reconstruction of different algorithms on WHU-RS19 dataset. (a) HR; (b) Bicubic; (c) SRGAN; (d) EDSR; (e) ESRGAN; (f) proposed algorithm
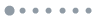
Fig. 8. Image reconstruction of different algorithms on AID dataset. (a) HR; (b) Bicubic; (c) SRGAN; (d) EDSR; (e) ESRGAN; (f) proposed algorithm
|
Table 1. Performance of algorithm on Kaggle test dataset under different module settings
|
Table 2. Performance of algorithm on Kaggle test datasets with different loss function settings
|
Table 3. Average PSNR of different algorithms on Kaggle, WHU-RS19, and AID
|
Table 4. Average SSIM of different algorithms on Kaggle, WHU-RS19, and AID
|
Table 5. Average FSIM of different algorithms on Kaggle, WHU-RS19, and AID
|
Table 6. Running time of different algorithms on Kaggle, WHU-RS19, and AID

Set citation alerts for the article
Please enter your email address