Chunming Li, Lü Dayong, Songling Yuan. Camera Calibration Based on Improved Grey-Wolf Genetic Algorithm[J]. Laser & Optoelectronics Progress, 2024, 61(18): 1815004

Search by keywords or author
- Laser & Optoelectronics Progress
- Vol. 61, Issue 18, 1815004 (2024)
Note: This section is automatically generated by AI . The website and platform operators shall not be liable for any commercial or legal consequences arising from your use of AI generated content on this website. Please be aware of this.
Abstract
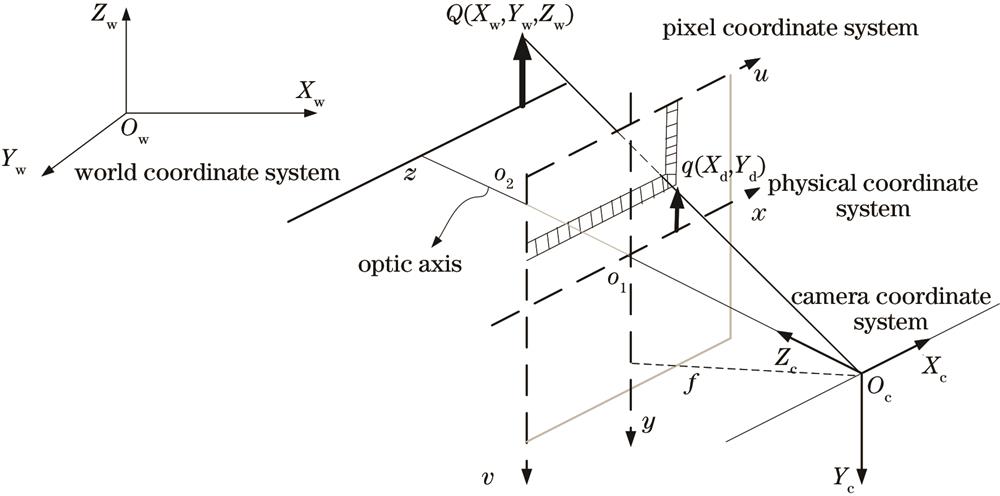
Set citation alerts for the article
Please enter your email address