Yu Huang, Yanlin Shao, Wei Wei, Qihong Zeng. Study on the Decision Tree Model for Carbonate Rock Lithology Identification Based on Hyperspectral Data[J]. Laser & Optoelectronics Progress, 2025, 62(8): 0837007

Search by keywords or author
- Laser & Optoelectronics Progress
- Vol. 62, Issue 8, 0837007 (2025)
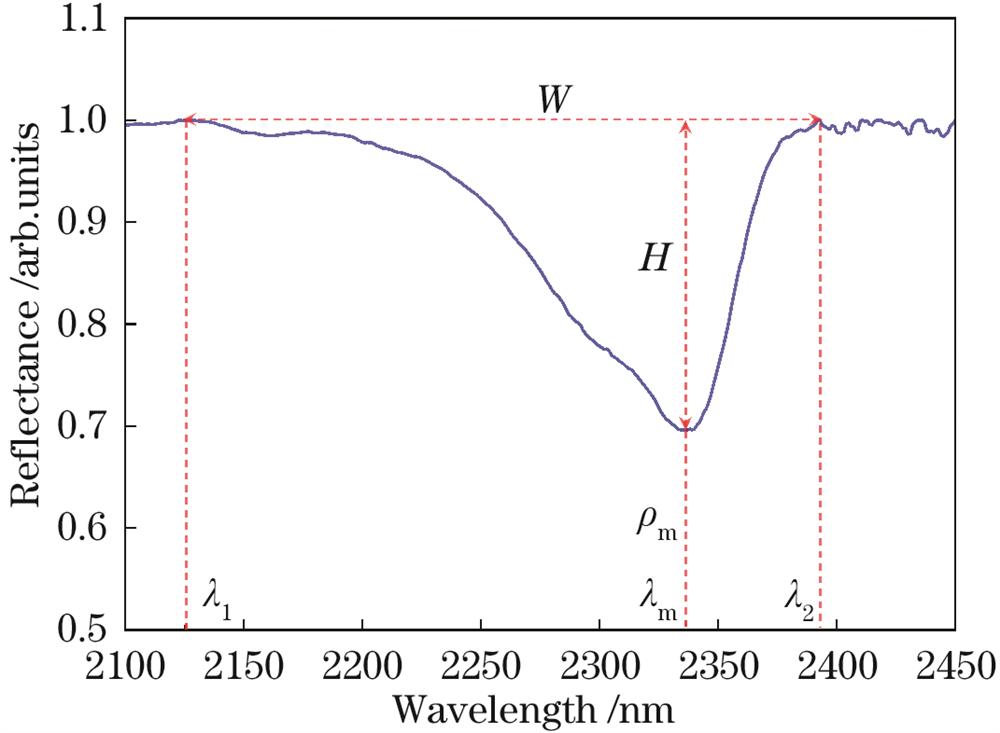
Fig. 1. Schematic diagram of spectral waveform features
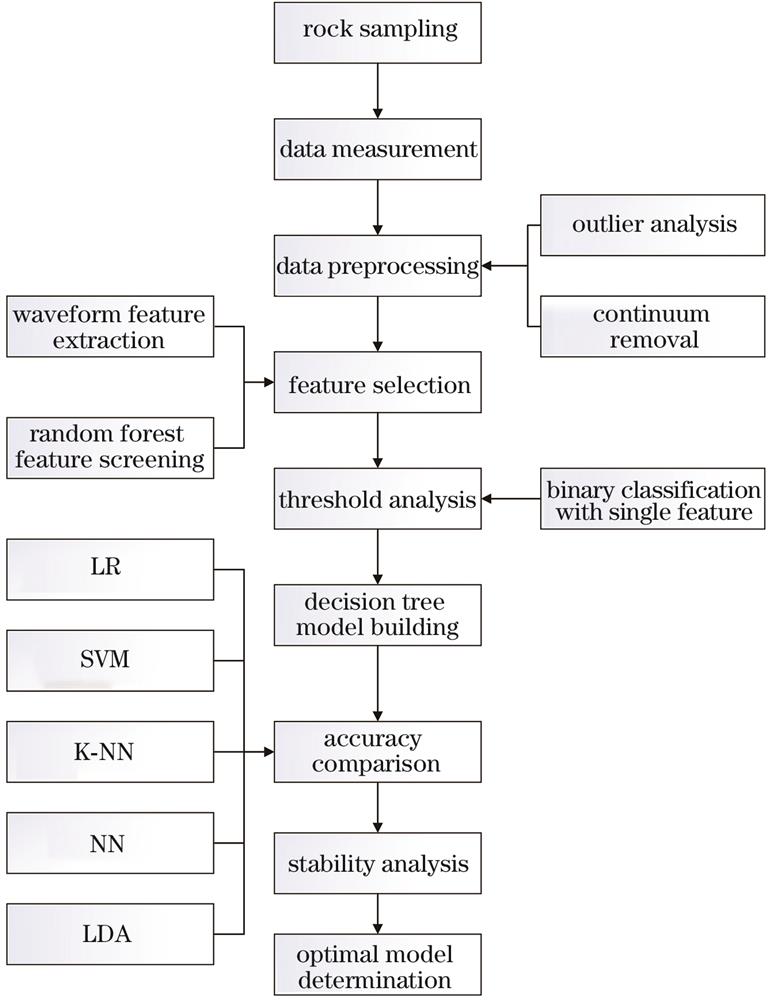
Fig. 2. Technology roadmap of proposed algorithm
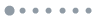
Fig. 3. Spectral curves before and after preprocessing
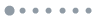
Fig. 4. Ranking of feature importance
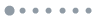
Fig. 5. Box plots of particial features. (a) Absorption bandwidth; (b) right shoulder wavelength of the absorption band; (c) the lowest point wavelength of the absorption valley
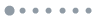
Fig. 6. Scatter plot of particial features
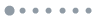
Fig. 7. Classification accuracy of different features at different thresholds. (a) Right shoulder wavelength of the absorption band and the lowest point wavelength of the absorption valley; (b) absorption bandwidth
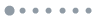
Fig. 8. Decision tree model accuracy under different feature combinations
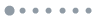
Fig. 9. Processing flowchart of decision tree
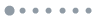
Fig. 10. Classification accuracy of different features for different test sets. (a) The lowest point wavelength of the absorption valley; (b) right shoulder wavelength of the absorption band; (c) absorption bandwidth
|
Table 1. Confusion matrix
|
Table 2. Comparison of accuracy among different models
|
Table 3. Feature importance under different test set proportions
|
Table 4. Confusion matrix by decision tree model
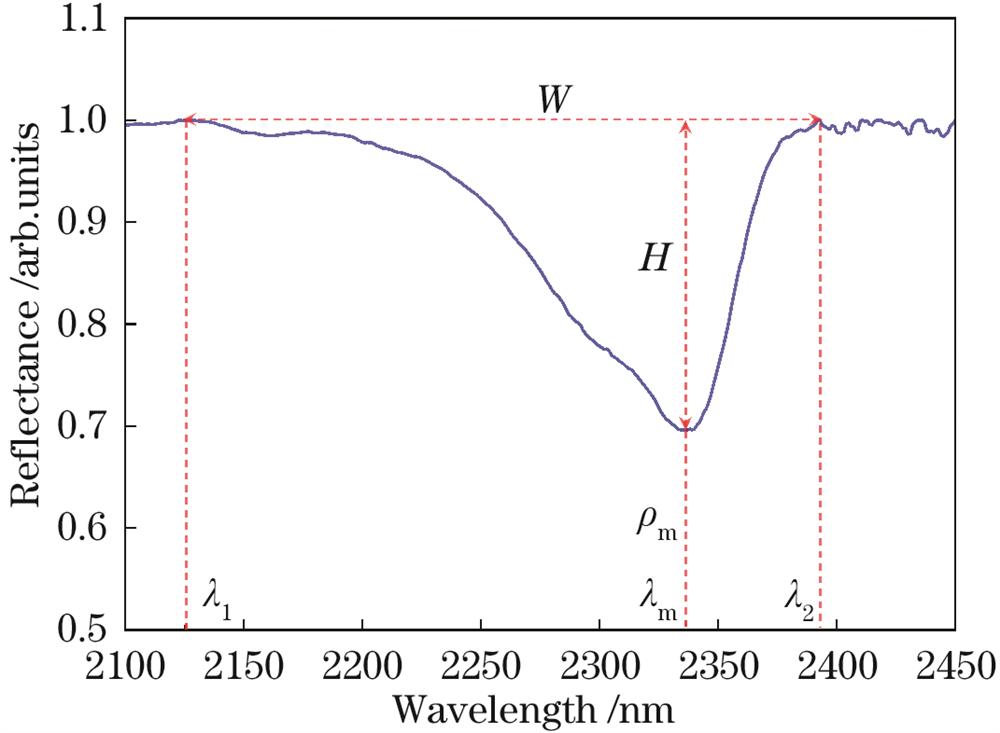
Set citation alerts for the article
Please enter your email address