NI Qin-ru, OU Quan-hong, SHI You-ming, LIU Chao, ZUO Ye-hao, ZHI Zhao-xing, REN Xian-pei, LIU Gang. Diagnosis of Lung Cancer by Human Serum Raman Spectroscopy Combined With Six Machine Learning Algorithms[J]. Spectroscopy and Spectral Analysis, 2025, 45(3): 685

Search by keywords or author
- Spectroscopy and Spectral Analysis
- Vol. 45, Issue 3, 685 (2025)
Abstract
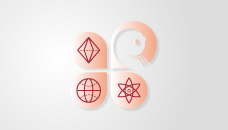
Set citation alerts for the article
Please enter your email address