Ziqi Han, Qiaohong Liu, Chen Ling, Jiawei Liu, Cunjue Liu. Polyp Segmentation Method Combining HarDNet and Reverse Attention[J]. Laser & Optoelectronics Progress, 2023, 60(2): 0217003

Search by keywords or author
- Laser & Optoelectronics Progress
- Vol. 60, Issue 2, 0217003 (2023)
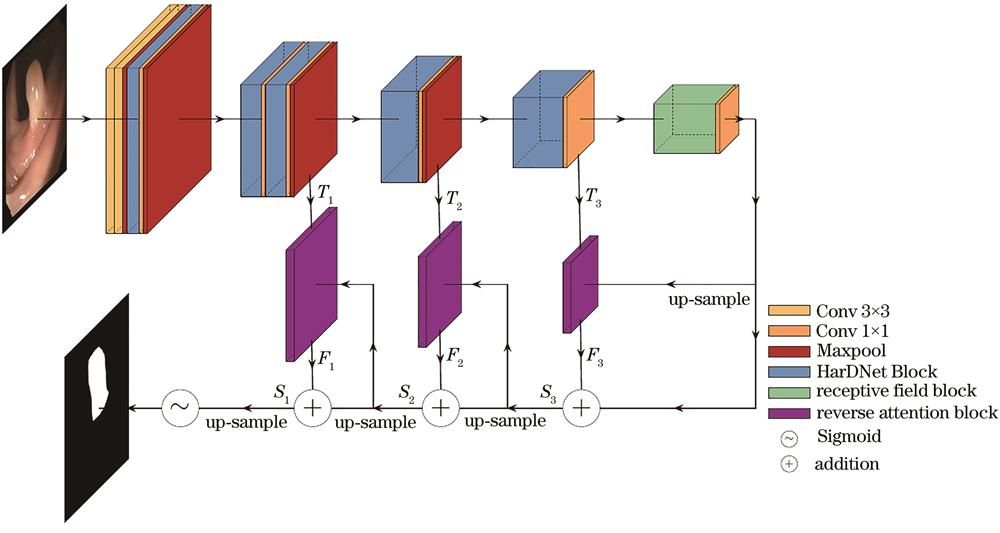
Fig. 1. HraNet model structure
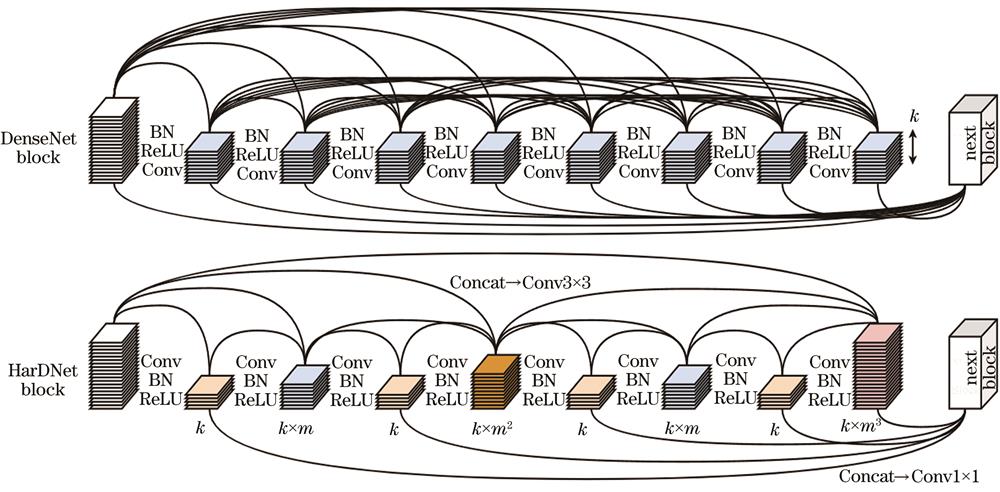
Fig. 2. Structure comparison diagram of DenseNet and HarDNet
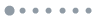
Fig. 3. Reverse attention block
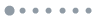
Fig. 4. Receptive field block
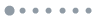
Fig. 5. Comparison of segmentation results of colonic polyps
|
Table 1. Detailed implementation parameters of HarDNet68
|
Table 2. Comparison of segmentation effects of different methods on Kvasir SEG and CVC ClinicDB datasets
|
Table 3. Generalization capability test results
|
Table 4. Comparison of training time and reasoning speed of different methods based on same platform
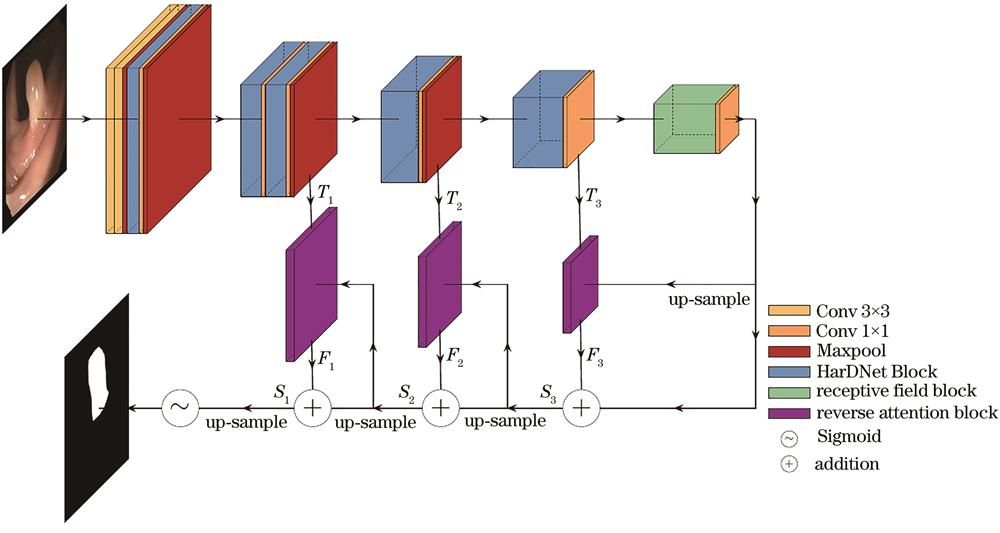
Set citation alerts for the article
Please enter your email address