Yangyundou Wang, Hao Wang, Min Gu. High performance “non-local” generic face reconstruction model using the lightweight Speckle-Transformer (SpT) UNet[J]. Opto-Electronic Advances, 2023, 6(2): 220049

Search by keywords or author
- Opto-Electronic Advances
- Vol. 6, Issue 2, 220049 (2023)

Fig. 1. SpT UNet architecture for spatially dense feature reconstruction (a ) with the multi-head attention (or cross attention) module (b ) included transformer encoder block (c ) and decoder block (d ).

Fig. 2. The puffed downsampling - module architecture.
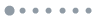
Fig. 3. The leaky upsampling - module architecture.
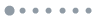
Fig. 4. Experiment set-up.
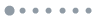
Fig. 5. Overview of the data acquisition under various conditions and the training/testing/validation of the SpT UNet. (a ) The training/testing data set is captured at T1 (0 mm), and T2 (20 mm). And the validation data set is captured at T3 (40 mm). The training/testing stage (b ) and the validation stage (c ) of the SpT UNet for the speckle reconstruction of the generic face images.
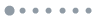
Fig. 6. The ground truth (left column) and prediction (right column) of the trained SpT UNet with the camera placed at 40 mm away from the focal plane. The prediction results are overlaid with the true positive (white), false positive (green), and false negative (red).
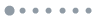
Fig. 7. Quantitative analysis of the trained SpT UNet using NPCC as the loss function (a ) and SSIM as the indicator for accuracy (b ).
|
Table 0. Performance of the SpT UNet.
|
Table 0. The validation performance of the trained SpT UNet.
|
Table 0. The comparison of the SpT UNet, ViT, and SWIN transformer on parameter numbers.

Set citation alerts for the article
Please enter your email address