Anna Wirth-Singh, Jinlin Xiang, Minho Choi, Johannes E. Fröch, Luocheng Huang, Shane Colburn, Eli Shlizerman, Arka Majumdar, "Compressed meta-optical encoder for image classification," Adv. Photon. Nexus 4, 026009 (2025)

Search by keywords or author
- Advanced Photonics Nexus
- Vol. 4, Issue 2, 026009 (2025)

Fig. 1. Schematic of CNNs for image classification tasks. (a) All-electronic multi-layered CNN. (b) All-electronic compressed CNN. (c) Hybrid CNN that combines an optical meta-optic front end and electronic backend. (d) Number of MAC operations of each network configuration, with convolutional MACs in green and fully connected MACs in brown.

Fig. 2. Schematic of the optical system. (a) PSF measurement setup using a monochromatic point light source (left) and optical convolution measurements using a micro-LED display (right). (b) Photograph of the fabricated meta-optics. The meta-optic contains 16 different suboptics, spatially distributed in a single layer, operating in parallel for classification tasks. (c) Phase maps and SEM images of exemplary suboptics corresponding to the positive and negative parts of a particular convolutional kernel. (d) Positive and negative parts of an example convolutional kernel (left), the corresponding PSF simulation (middle), and experiment (right). (e) Simulated electronic output (left) and optical experiment (right) convolved output for the example kernel, for the case of an input “7” from MNIST.
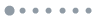
Fig. 3. Confusion matrices for different network architectures. (a) Classification results for AlexNet-Mod (multiple-layer electronic CNN). (b) Classification results for the all-electronic CNN compressed without using KD. (c) Classification results for the all-electronic CNN compressed with KD. (d) Classification results for the hybrid optical–electronic CNN.
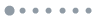
Fig. 4. PCA of the hybrid CNN. (a) PCA of the uncalibrated experimental hybrid CNN classification data. (b) PCA of the calibrated experimental data, which has been remapped and exhibits clustering behavior similar to that of the compressed electronic CNN data. (c) PCA of the compressed electronic CNN data.
|
Table 1. Classification results.
|
Table 2. Network ablation study.

Set citation alerts for the article
Please enter your email address